FEDS Notes
October 21, 2020
Electricity Demand as a High-Frequency Economic Indicator: A Case Study of the COVID-19 Pandemic and Hurricane Harvey
Joshua Blonz and Jacob Williams
Intro
Electricity is used by all businesses in the United States. During quickly moving economic shocks—for example, a pandemic or natural disaster—changes in electricity consumption can provide insight to policymakers before traditional survey-based metrics, which can lag weeks or months behind economic conditions and typically only show a snapshot of when the survey was conducted. While traditional indicators can give a more comprehensive view of the economy, it is precisely during periods of rapid economic changes that the shortcomings of traditional indicators make high-frequency (HF) indicators valuable.
In this note, we explore the potential value of electricity consumption data as an HF indicator in a variety of settings. Several studies have explored the link between electricity usage and economic activity, including research by Arora and Lieskovsky (2014) that found a strong correlation between growth rates in real gross domestic product and electricity use. This research generally used monthly or annual electricity usage data, which were released several weeks or months after the usage period. In recent years, however, electricity demand data has increasingly become available in real time due to the wide-scale adoption of smart meter infrastructure. While electricity demand is not a substitute for traditional metrics, it can provide additional insight at a finer temporal and geographic scale. We focus on two main advantages of electricity indicators.
First, electricity-based indicators are able to track changes in economic activity in near real time, which is useful during downturns and recoveries that may never be captured by traditional data. For example, quantifying the short-duration shutdowns and recoveries from hurricanes in traditional statistics is a challenge in real time and may never be fully measured. Electricity consumption is also available at a relatively fine geographic scale, which can be useful in evaluating regional shocks.
Second, electricity demand is a widely available indicator that can be used across all sectors of the economy. Many HF indicators can provide precise measures on a specific portion of the economy (for example, OpenTable reservations for restaurant demand) but lack coverage for other industries. In particular, there is a lack of HF indicators for large industrial customers that electricity demand can help inform.
Despite their advantages, electricity indicators must be interpreted with caution. The relationship between electricity usage and economic output can shift in unknown ways during a severe shock, making it challenging to directly translate changes in electricity demand to economic activity. Given this challenge, electricity HF indicators are best used to determine when economic activity began to decline, when the recovery starts and progresses, and when demand has returned to pre-shock levels.
In this paper, we use electricity demand data in Texas to demonstrate the value of electricity as an HF indicator for two different economic shocks. First, we examine the effects of the COVID-19 pandemic on electricity demand using Texas grid-level data as well as controls for seasonal patterns and outdoor temperature to show aggregate deviations in demand from expected "normal" usage. We then use electricity consumption by customer type to show how residential, small commercial and industrial (C&I), and large C&I customers each responded differently during the first four months of the pandemic. We find that while overall Texas demand fell as much as 8 percent in April, it recovered to almost normal levels in July and August. In contrast, as of August, residential demand remains around 8 percent above normal and large C&I demand remains 10 percent below normal. Different recovery patterns can be seen regionally: The Dallas region had recovered to almost normal levels by late July, but the west Texas region remains 17 percent below normal.
We also consider the potential value of our electricity HF indicator on a more frequent economic shock: hurricanes. We find that electricity demand can be a useful HF indicator that can help determine when economic activity returns to pre-hurricane levels. Breaking down the effects by region and customer type provides additional insight on the timing and geography of the economic effects.
This note explores the potential value of electricity as an HF indicator. Our findings highlight that electricity demand can be helpful, but it is more useful when disaggregated data are available for analysis. Only using the Texas-wide aggregate indicator shows that the Texas economy has mostly recovered from the economic effects of COVID-19 by early August. However, the disaggregated data tell a more nuanced story of large C&I customers that have a sustained below-normal demand that has not improved over the first four months of the pandemic, highlighting the value of the electricity indicator when broken out by customer type.
Data Description
Hourly electricity consumption data by weather zone and customer class is collected from the Electric Reliability Council of Texas (ERCOT) from 2010 to the present. ERCOT manages the flow of electric power for 90 percent of Texas's electricity demand.1 The electricity consumption data represent system load, which is defined as the amount of power drawn from the electric grid as measured by energy meters. These data are available with a few days lag directly from ERCOT.
Hourly air temperature data are collected from the Iowa Environmental Mesonet's archive of automated airport weather station observations. We use 186 stations across Texas and population weight average hourly temperature up to either the grid region or the full ERCOT service territory. The weighted temperature readings are used to calculate cooling and heating degree days.
Methodology
The goal of our econometric analysis is to determine the deviation in demand from previous years conditional on outdoor temperature, hour of day, day of week, and other relevant factors such as holidays. We estimate this deviation using the following regression:
(1) ln(loadwdh)=βw+δd+γh+hdwdh+cdwdh+holidaywdh+εwdh,
Where ln(loadwdh) is the log of electricity load on week of year w, day of week d, and hour of day h. βw are the coefficients of interest and are estimated for each week of the year. The coefficients are only estimated for the final year of the sample and compare a given week to that same week in previous years, similar to the methodology in Cicala (2020). δd is a day-of-week fixed effect and γh is an hour-of-day fixed effect. hdwdh and cdwdh are heating and cooling degree days, respectively, which we calculate at the hourly level, and holidaywdh controls for federal holidays. εwdh is the error term, which is clustered at the date-of-sample level.
The βw coefficients represent the percent change in demand in week of year w relative to what would be expected given the observable temperature and demand characteristics. Similar to Cicala (2020), we normalize coefficients relative to the pre-period (February 2020 for studying COVID-19) as a baseline to make the interpretation easier.
Statewide Effects of COVID-19 Pandemic
Figure 1 shows the effects of COVID-19 on electricity demand using publicly available data from ERCOT. The hollow dots are regression coefficients that show the weekly deviation in demand from normal levels estimated in equation 1. The vertical capped bars indicate 95 percent confidence intervals. We use a number of gray and red vertical lines to indicate social-distancing policies designed to reduce the spread of COVID-19. The leftmost vertical gray dashed line shows when Austin, Dallas, and Houston enacted stay-at-home orders on March 24. Between April 2 and April 30, a statewide stay-at-home order was in place, which is shown by the two solid red lines on each panel. Restaurants, retail stores, and movie theaters were allowed to reopen indoor service on May 1, with capacity limited to 25 percent.2 On May 18, childcare centers, gyms, and nonessential manufacturing facilities were allowed to reopen as Texas entered phase two. Starting June 3, all businesses were allowed to operate at up to 50 percent capacity as part of phase three of reopening, and restaurants were allowed to operate at up to 75 percent capacity starting June 12. On June 26, however, Governor Abbott ordered bars to close and scaled back restaurant capacity to 50 percent amid a surge in COVID-19 cases, which we show with the far right dashed red line.
Panel A of figure 1 shows the effects of COVID-19 on total electricity demand in Texas. The results show a 4 to 8 percent reduction in demand for all customers in the first couple months of the pandemic, which suggests a decline in aggregate economic activity. Demand is furthest below normal during the statewide shutdown in April and increases a small amount once the stay-at-home order is ended across the state and the first phase of reopening begins on May 1. The June 26 scale back does not seem to have an effect on aggregate electricity consumption.
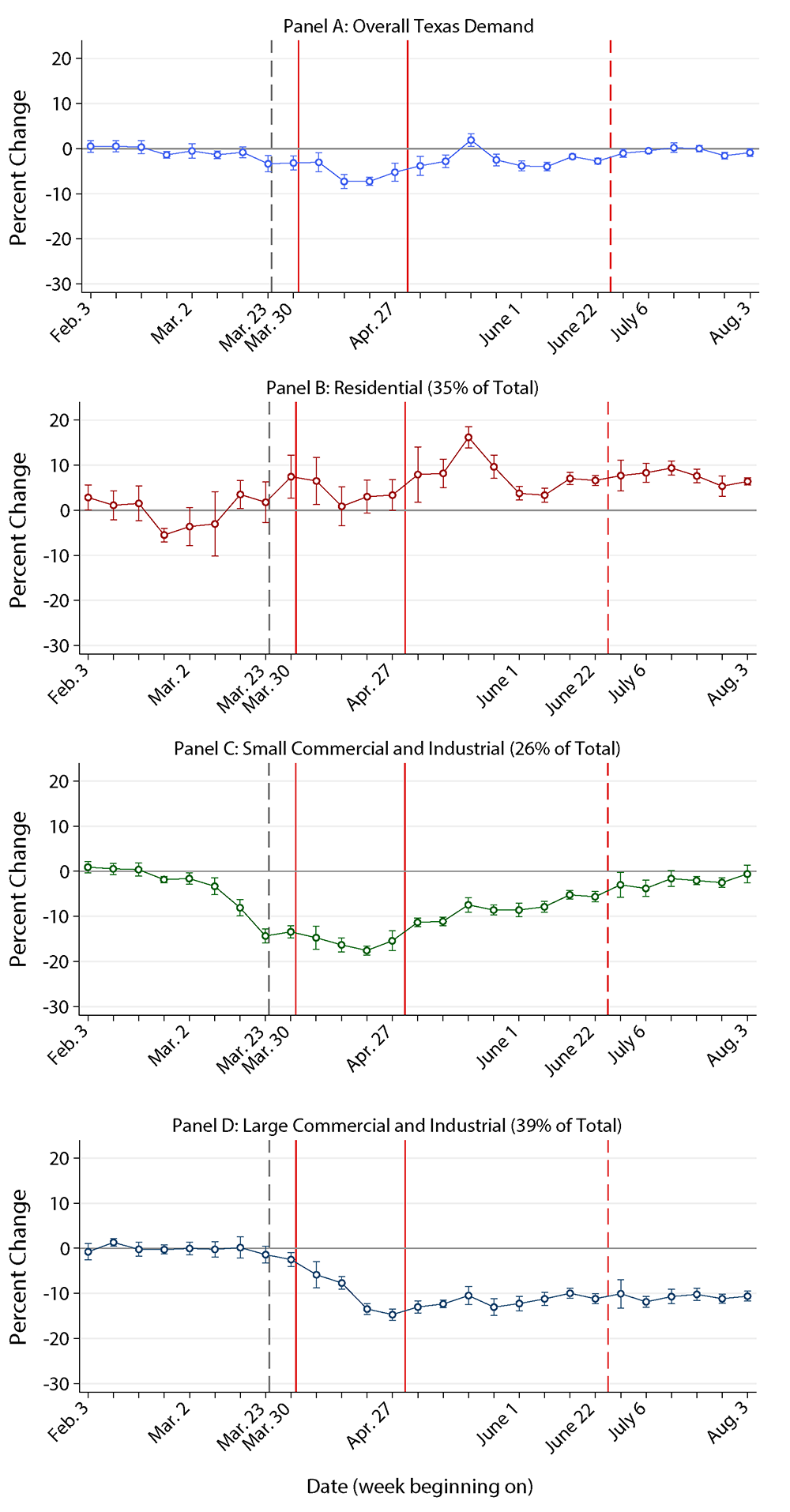
Note: Dots represent weekly deviation from demand from the same week of the year in previous years conditional on outdoor temperature and fixed effects. Results are normalized based on the average coefficient values in February 2020 for ease of interpretation. The vertical capped bars indicate the 95 percent confidence interval. The dashed gray line shows when local stay-at-home orders went into effect in Houston, Dallas, and Austin on March 24. The solid red lines show when the state-wide stay-at-home order was in place between April 2 and April 30. The dashed red line shows when Governor Abbot ordered bars to close again effective on June 26. Phased reopening began May 1 and continued through June.
Source: Electric Reliability Council of Texas.
Panels B through D break out the results by residential, small C&I, and large C&I groupings, respectively.3 Residential customers, shown in panel B, make up around 35 percent of demand, and their electricity usage appears to be mostly increasing during the pandemic. This finding reflects the stay-at-home orders imposed by state and local authorities as well as the social-distancing practices recommended by public health officials during the pandemic. In contrast, small C&I customers, shown in panel C, decreased consumption by as much as 17 percent during the stay-at-home order. Small C&I, which make up 26 percent of demand, include restaurants and retail establishments, and the reduction in demand likely reflects lower customer demand as well as government-mandated business closures. The slow recovery in small C&I electricity demand in May, June, and July likely reflects the increased economic activity during the phased reopening. The reimposition of limited restrictions in late July does not appear to have affected small C&I demand.
Panel D shows large C&I customers, which make up 39 percent of demand and include the biggest electricity users in Texas. The pattern of decline differs, with major reductions happening in April followed by a sustained level of depressed demand through early August. This trend suggests that large businesses were affected differently by the COVID-19 pandemic than other parts of the Texas economy, and that operations for some large users have not recovered to pre-pandemic levels.
Panels A and B of figure 2 break out the results for the north central (including Dallas) and far west (including Midland) regions of the ERCOT service territory. The north central region makes up around 32 percent of Texas demand, and its electricity usage declined during the statewide shutdown in April and then gradually returned close to normal by early July. In the far west region, electricity usage slowly declined, reaching more than 20 percent below normal in late May. Demand recovered slightly in early June and remains around 15 percent below normal in July and August. The far west region makes up around 7 percent of Texas demand and has many oil fields, including some that stopped drilling new wells and suspended production at existing wells when oil prices plunged in March and April, which likely accounts for part of the depressed electricity usage in the region.
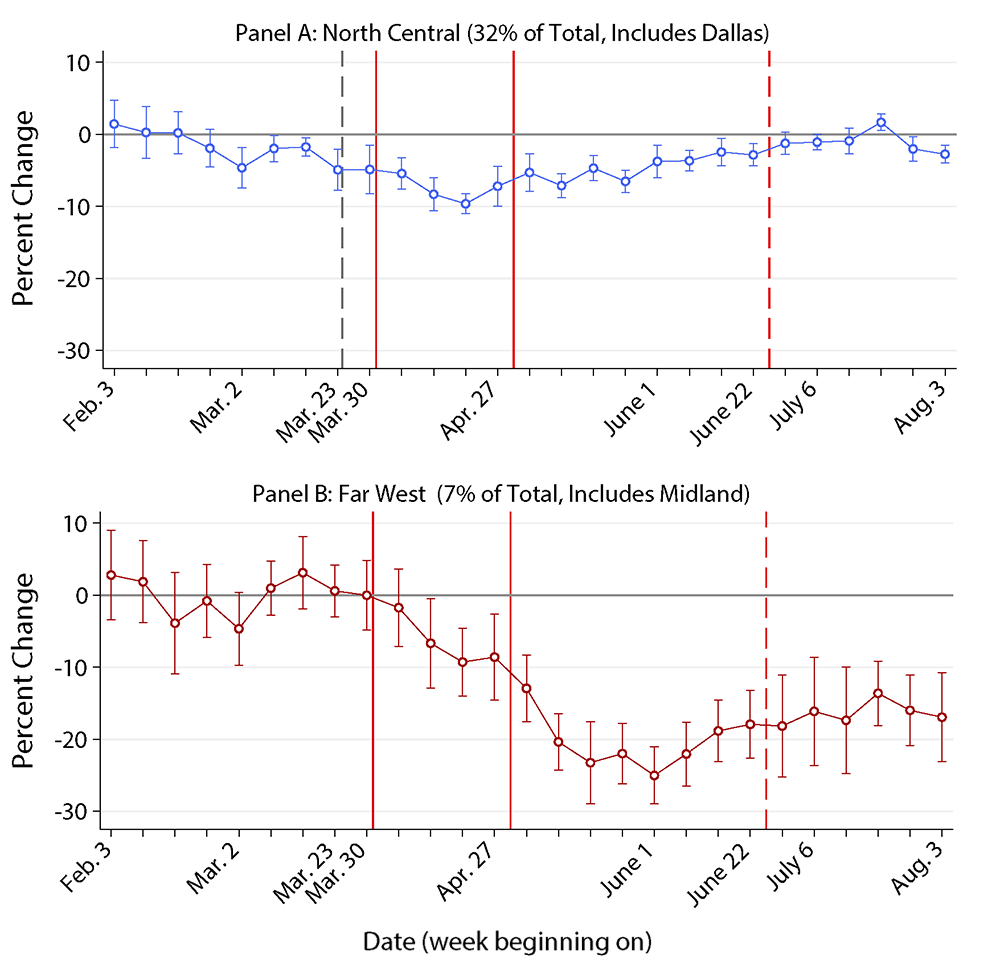
Note: Dots represent weekly deviation from demand from the same week of the year in previous years conditional on outdoor temperature and fixed effects. Results are normalized based on the average coefficient values in February 2020 for ease of interpretation. The vertical capped bars indicate the 95 percent confidence interval. The dashed gray line in panel A shows when a local stay-at-home order went into effect in Dallas on March 24. The solid red lines show when the state-wide stay-at-home order was in place between April 2 and April 30. The dashed red line shows when Governor Abbot ordered bars to close again effective on June 26. Phased reopening began May 1 and continued through June.
Source: Electric Reliability Council of Texas.
Taken together, it is possible to see that the decline of 4 to 8 percent in overall Texas electricity usage followed by the return to pre-pandemic levels in July and August masks important heterogeneity seen in different sectors and regions. Aggregate Texas demand suggests that electricity demand, and by extension economic activity, has mostly recovered to normal levels. However, the sector-level data tells a different story. Small C&I customers have mostly recovered, but large C&I customers remain around 10 percent below expected levels, while residential demand is a little less than 10 percent above expected levels. Similarly, some regions have recovered, while others in western Texas have demand far below normal levels. These detailed results highlight the value of sector and regional electricity indicators.
Potential Application for Hurricanes
Electricity is well suited as an HF indicator for rapidly moving crises such as hurricanes or floods. Many traditional survey statistics are not able to capture short-lived events. Credit card transaction data have been used to track retail activity during other hurricanes, but they do not provide much insight on the industrial sector (Aladangady and others, 2017). In this section, we explore the potential value of electricity during Hurricane Harvey, which caused widespread damage in the Houston area. As a case study, we focus on the potential value that an electricity-based indicator could provide to the Federal Reserve's G.17 release on industrial production (IP) and capacity utilization.4
IP was one of the first estimates of the effect of Hurricane Harvey on economic activity. Data on production-worker hours from the Current Employment Statistics program at the Bureau of Labor Statistics (BLS), a key component of IP, were unavailable for most industries, however, as the data come from a BLS survey covering the pay period containing the 12th of the month. Because Hurricane Harvey hit Texas at the end of the month, the production-worker hours for August did not show an effect of the disruption and would have overstated economic activity during the month. Bayard, Decker, and Gilbert (2017) describe the "natural disaster" procedure used to adjust the monthly IP indexes for those industries (about 40 percent of IP that relies primarily on production worker hours) to accurately reflect the substantial disruptions in activity. The procedure involves assigning a severity rating to counties affected by Hurricane Harvey and then using the ratings in conjunction with county employment data to estimate how the relatively short-lived Hurricane Harvey reduced total national IP.
Central to the disruption estimates is the timing of the hurricane. It is possible to use hurricane landfall as a starting point, but this may miss anticipatory closures and longer-run consequences of the storm. Electricity usage is well positioned to supplement the determination of when the natural disaster began and ended. It also can provide some insight on the severity of its impact by region and industry.
Figure 3 presents the main regression results for August and September 2017. We use the same specification as equation 1 but estimate coefficients at the daily rather than the weekly level along with adding a week-of-year fixed effect. The solid red lines indicate when Hurricane Harvey first made landfall in Texas on August 25, 2017 (around 10 p.m. local time) and dissipated further inland on August 31, 2017. They nearly line up with the five-day period from August 26, 2017, to August 30, 2017, that industries were assumed to be shut down as part of the natural disaster procedure used in calculating IP.

Note: Dots represent daily deviation from demand from the same day of the year in previous years conditional on outdoor temperature and fixed effects. Results are normalized based on the average coefficient values in July 2017 for ease of interpretation. The vertical capped bars indicate the 95 percent confidence interval. The solid red lines indicate when Hurricane Harvey first made landfall in Texas on August 25 and dissipated further inland on August 31.
Source: Electric Reliability Council of Texas.
Panel A shows Texas-wide electricity demand, which dropped by about 15 percent after Hurricane Harvey hit. Panel B shows large C&I; electricity consumption drops sharply, reflecting the sector's concentration in the heavily affected coastal region of Texas, but it takes several weeks before consumption reaches its pre-hurricane levels. Oil refineries, which are included in large C&I, were hard hit, and many either reduced operations or shut down for weeks afterward (Egan, 2017). Panel C shows the coast region (including Houston). Panel D shows the west region (including Abilene). There is a much smaller decline over that period for the other regions (not shown).
While the decline in electricity usage begins around the same time for each region and industry, there is substantial variation in when each fully recovers. The less affected west region returns to pre-hurricane electricity usage in early September, while the heavily affected coast region does not return to normal usage until mid-September. Large business electricity usage also does not return to normal until mid-September. Bayard, Decker, and Gilbert (2017) find a similarly long recovery path in industries such as oil refining, plastic resins, and organic chemicals where weekly data are available. Our large business electricity index includes those industries as well as many others where detailed weekly data are not available. The electricity data thus could provide additional insights into the recovery path of large businesses, which is a crucial but usually difficult-to-obtain piece of information used to calculate IP. This type of analysis could potentially be helpful for estimating IP during future natural disasters.
Limitations of Electricity as a High-Frequency Indicator
This note shows the potential uses of electricity as an HF economic indicator. It is unique in its ability to provide HF data with relatively full coverage of economic activity. Creating a high-level indicator such as panel A of figure 1 is currently possible throughout the United States. However, there are a number of logistical hurdles that may prevent the disaggregated indicators from being universally available.
First, smart meters, which allow for real-time collection of household or establishment-level consumption, are important for estimates below the grid level. The ERCOT breakdown by customer class in figure 1 requires large numbers of smart meters; smart meters now make up around 50 percent of meters in the United States (Federal Energy Regulatory Commission, 2019). Some states, such as California, Texas, and Georgia, have almost 100 percent smart meter penetration, while other states, including New York and New Jersey, have few smart meters. The number of smart meters used nationwide continues to increase around 10 percent per year (Cooper and Shuster, 2019). Eventually, smart meters will be installed in most establishments and homes in the United States (for example, New York City is on track to have all smart meters by 2022).5
Second, the ERCOT data by customer type do not exist in every region of the country. The data are limited by the penetration of smart meters and other institutional factors. Moreover, many grid operators may not want or need to release data by customer type or sector, and their reach is partially limited by various grid structures and rules. Over time, as smart meter usage increases, more of this data will likely be made available by grid operators or for purchase.
Finally, the U.S. grid is not one unified grid but rather 66 different grids named balancing authorities (Hoff, 2016). Data on grid-level consumption is recorded for all U.S. grids by the U.S. Energy Information Administration, but the Energy Information Administration only tracks aggregate consumption and does not include any of the disaggregated categories discussed in this paper. As a result, even if disaggregated data are available, such data must be collected grid by grid.
Future Applications
The barriers preventing the widespread use of electricity as an HF indicator are not insurmountable and are shrinking over time. This note has shown that electricity HF indicators can have value in tracking the COVID-19 pandemic and in supplementing traditional data during natural disasters. As smart meter penetration increases in the United States, similar indicators will become available in more regions of the country.
In areas where smart meters are widely installed, there is an opportunity for local regulators and policymakers to work in partnership with electric utilities to develop real-time indicators at the industry level. It would be relatively easy for a utility to produce a real-time data stream of electricity demand by customer type and region. For example, a utility could provide electricity consumption data for large food processors, which could be turned into a real-time utilization index. Such an index could be provided confidentially to state regulators, local policymakers, or regional Federal Reserve Banks to improve real-time analysis by giving highly detailed industry-level performance. Access to this disaggregated data would also improve the ability to determine how changes in electricity demand correlate with changes in economic activity, allowing for the improvement of electricity as an HF indicator over time. With the correct data infrastructure in place, HF electricity indicators could be available to policymakers for the next crisis.
References
Aladangady, Aditya, Shifrah Aron-Dine, Wendy Dunn, Laura Feiveson, Paul Lengermann, and Claudia Sahm (2016). "The Effect of Hurricane Matthew on Consumer Spending," FEDS Notes. Washington: Board of Governors of the Federal Reserve System, December 2.
Arora, Vipin, and Jozef Lieskovsky (2014). "Electricity Use as an Indicator of U.S. Economic Activity (PDF)," Working Paper Series. Washington: U.S. Energy Information Administration, November.
Bayard, Kimberly, Ryan Decker, and Charles Gilbert (2017). "Natural Disasters and the Measurement of Industrial Production: Hurricane Harvey, a Case Study," FEDS Notes. Washington: Board of Governors of the Federal Reserve System, October 11.
Cicala, Steve (2020). "Early Economic Impacts of COVID-19 in Europe: A View from the Grid (PDF)," working paper. Chicago: University of Chicago, April 8.
Cooper, Adam, and Mike Shuster (2019). "Electric Company Smart Meter Deployments: Foundation for a Smart Grid (2019 Update) (.ashx)." Washington: Edison Foundation Institute for Electric Innovation, December.
Egan, Matt (2017). "Texas Oil Refineries Still Badly Hurting from Harvey," CNN Business, September 11.
Federal Energy Regulatory Commission (2019). "2019 Assessment of Demand Response and Advanced Metering (PDF)," staff report. Washington: Federal Energy Regulatory Commission, December.
Hoff, Sara (2016). "U.S. Electric System Is Made Up of Interconnections and Balancing Authorities." Washington: U.S. Energy Information Administration, July 20.
Kurmann, André, Etienne Lalé, and Lien Ta (2020). "The Impact of COVID-19 on Small Business Employment and Hours: Real-Time Estimates With Homebase Data (PDF)," working paper. Philadelphia: Drexel University, May 4.
1. For more details, see http://www.ercot.com/content/wcm/lists/197391/ERCOT_Fact_Sheet_6.1.20.pdf. For a map of the ERCOT service territory weather zones, see http://www.ercot.com/content/wcm/landing_pages/89373/ercotWeatherZoneMap.png. Return to text
2. Governor Abbot prevented local authorities from imposing further restrictions on activity after May 1. Return to text
3. Only 74 percent of ERCOT demand can be broken out into these three categories due to regulatory reasons. The remaining 26 percent is not reflected in panels B through D, but it follows the same pattern as total demand in panel A. The percent of demand for each category reported in the text reflects the percent of demand that can be broken out into the major categories. Return to text
4. The IP estimates for the electric utility industry are ultimately based on monthly data on national generation and sales from the U.S. Energy Information Administration once they become available; the initial estimates, however, reflect weekly data on electricity generation from the Edison Electric Institute. Up through 2005, monthly electricity demand for manufacturing industries at the 3-digit SIC/4-digit NAICS level and the district level was provided to IP estimators by the regional Federal Reserve Banks. In 2005, about 20 IP indexes were estimated based on these data, but unfortunately, the sample had deteriorated over time, leading to their retirement from IP at the end of that year. Return to text
5. For a map of smart meter penetration in New York City, see https://www.coned.com/en/our-energy-future/technology-innovation/smart-meters/when-will-i-get-my-smart-meter. Return to text
Blonz, Joshua, and Jacob Williams (2020). "Electricity Demand as a High-Frequency Economic Indicator: A Case Study of the COVID-19 Pandemic and Hurricane Harvey," FEDS Notes. Washington: Board of Governors of the Federal Reserve System, October 21, 2020, https://doi.org/10.17016/2380-7172.2781.
Disclaimer: FEDS Notes are articles in which Board staff offer their own views and present analysis on a range of topics in economics and finance. These articles are shorter and less technically oriented than FEDS Working Papers and IFDP papers.