FEDS Notes
December 03, 2021
New Accounting Framework Faces Its First Test: CECL During the Pandemic
Bert Loudis, Sasha Pechenik, Ben Ranish, Cindy M. Vojtech, and Helen Xu1
Introduction
On January 1, 2020, most large and mid-sized U.S. banks adopted Current Expected Credit Losses (CECL), a new accounting standard for estimating allowances.2 Allowance for credit losses is an estimate of the amount that a bank is unlikely to recover from a financial asset. CECL was issued to replace the incurred loss methodology (ILM) as policy makers determined that the increase in allowances occurred too late in the business cycle under ILM. By changing the timing and level of allowances, CECL also impacts the timing and level of capital, which could impact lending.
Generally, loan losses (also known as net charge-offs) build when unemployment increases and GDP slows.3 This relationship is clear during the 2007–09 financial crisis shown in Figure 1. Just a few months after CECL was adopted, the economy fell into a sharp decline as the COVID-19 pandemic resulted in widespread disruption to business activities (Figure 1, March 2020). This coincidence was an immediate test of the CECL methodology during an economic downturn and recovery, albeit an unusual one.
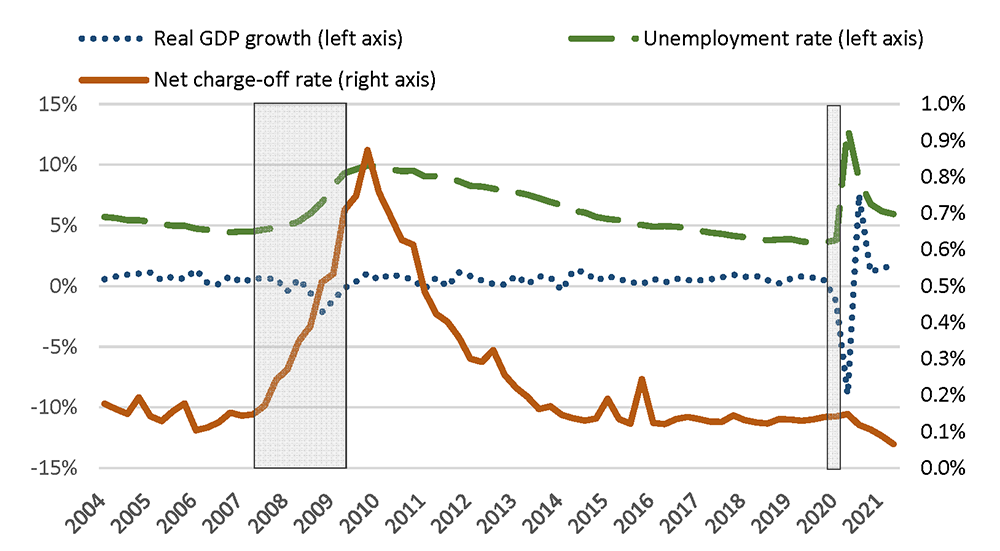
Note: Recessions are shaded in grey. The net charge-off rate is measured relative to total loans.
Sources: FRED, FR Y-9C, FFIEC Call Reports, and NBER.
When a bank builds allowances through provision expense, net income is reduced and that reduces capital. Consequently, as capital is generally considered a costly funding source, loans that carry larger allowances under CECL (as compared to ILM) become more expensive to underwrite.4 In response, banks may change their lending practices. This note estimates how CECL changed allowances and capital, and further explores the relationship between CECL and lending growth. Because only part of the banking system was initially subject to CECL, the analysis compares banks that adopted CECL on January 1, 2020 (CECL adopters) and banks that remained in ILM as of June 30, 2021 (non-adopters) to estimate these effects.5
Our analysis shows that CECL adopters' allowances responded more quickly than non-adopters to changes in the economic outlook. In addition, we show that while CECL increased aggregate allowances, the aggregate capital impact has been largely neutralized by the regulatory capital transition rule.6 We show that CECL has had a wide range of impact on allowances for different loan types. However, we find limited evidence that the impact of CECL on allowances is associated with decreased lending in the pandemic.
Background
ILM accounting standard limits banks' ability to provision for losses that are not yet probable. The financial crisis of 2007-2009 demonstrated significant problems with this limitation. In particular, banks had low levels of allowances to absorb credit losses in the lead up to the financial crisis. As the crisis unfolded, banks increased provisions for probable loan losses. This put additional pressure on bank capital, which was already being depleted due to other losses during the crisis.
Figure 2 shows this "too little, too late" relationship. Provisions (solid positive bars) sharply increase only after the recession has begun and net charge-offs increase (hollow negative bars). Provisions outpace net charge-offs to build allowances (black line), with allowances peaking around 2010, after the recession has ended.
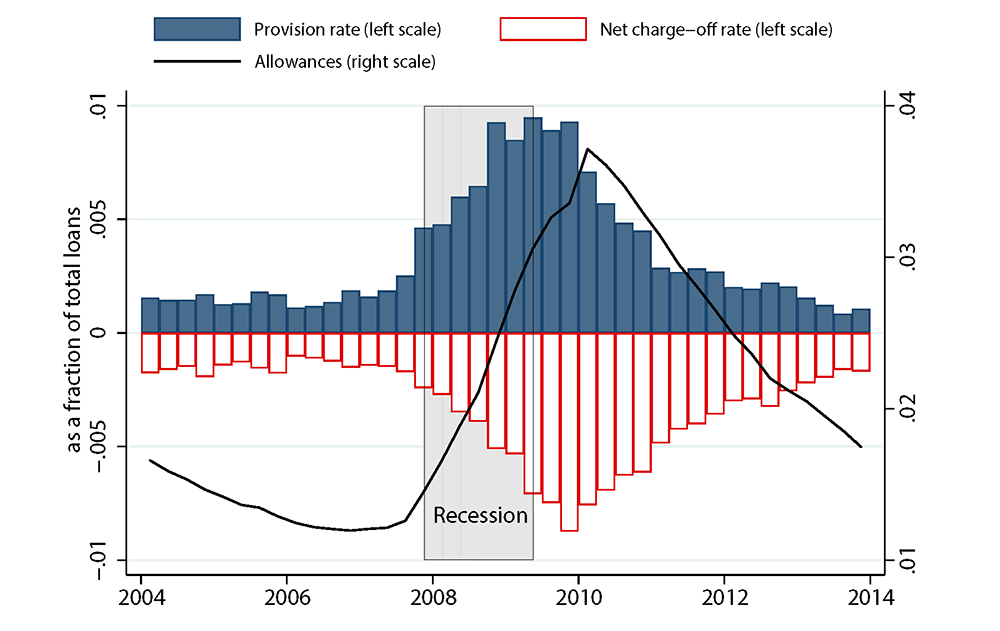
Note: The increase in allowance in 2010:Q1 reflects the introduction of FAS 166 and FAS 167 that tightened the accounting for securitizations and consolidation of securitization entities, respectively, causing banks to recognize securitized assets on the balance sheet.
Sources: FR Y-9C filing firms and Call Report firms with filings not consolidated within included filings.
To address this issue, various stakeholders requested that the accounting standard-setters, the FASB and International Accounting Standards Board, work to incorporate more forward-looking information in standards on loan loss provisioning. In response, the FASB issued a new accounting standard in June 2016 that introduced the CECL methodology for estimating allowances for credit losses.
In comparison to ILM, CECL requires banks to consider forward-looking information and record a provision for expected losses over the entire life of the loan when the loan is originated. CECL does not change the total amount of credit losses that will be ultimately recorded in financial statements, but it affects how early the bank provisions for credit losses. Because CECL allowances represent an estimate of lifetime losses—as opposed to losses over a typically shorter "loss emergence period" under ILM—CECL generally results in a higher level of allowances at each reporting period.
CECL's Impact on Allowances in the Pandemic
Due to the COVID-19 pandemic, many households and businesses faced a sudden loss of income. At the same time, extraordinary fiscal and monetary policy support blunted the financial stress. This section examines the differences in credit loss provisioning for CECL adopters and non-adopters during this unusual and highly uncertain environment.
Figure 3 compares the evolution of allowances for CECL adopters and non-adopters. Allowances of both sets of banks were stable in 2019, reflecting low and stable net charge-off rates. The adoption of CECL resulted in an immediate 37 percent increase in adopters' allowances on January 1, 2020. This impact represents an overall average difference in allowances between CECL and ILM under the generally benign economic outlook that existed on January 1, 2020. From that point onwards, the adoption of CECL and the onset of the pandemic both affected provisioning.
As the pandemic stressed the economy, CECL adopters rapidly increased loss provisions. Not counting the adoption impact, allowances increased by 76 percent in the first half of 2020 relative to 2019:Q4 levels. In comparison, non-adopters' allowances increased by only 32 percent over the same period. After peaking in 2020:Q2, CECL adopters' allowances begin to decline with improvement in the economic outlook. Non-adopters' allowances peaked in 2020:Q4, and have only declined slightly since then. As of 2021:Q2, the difference in allowances between CECL adopters and non-adopters is back to where it was immediately after CECL adoption.
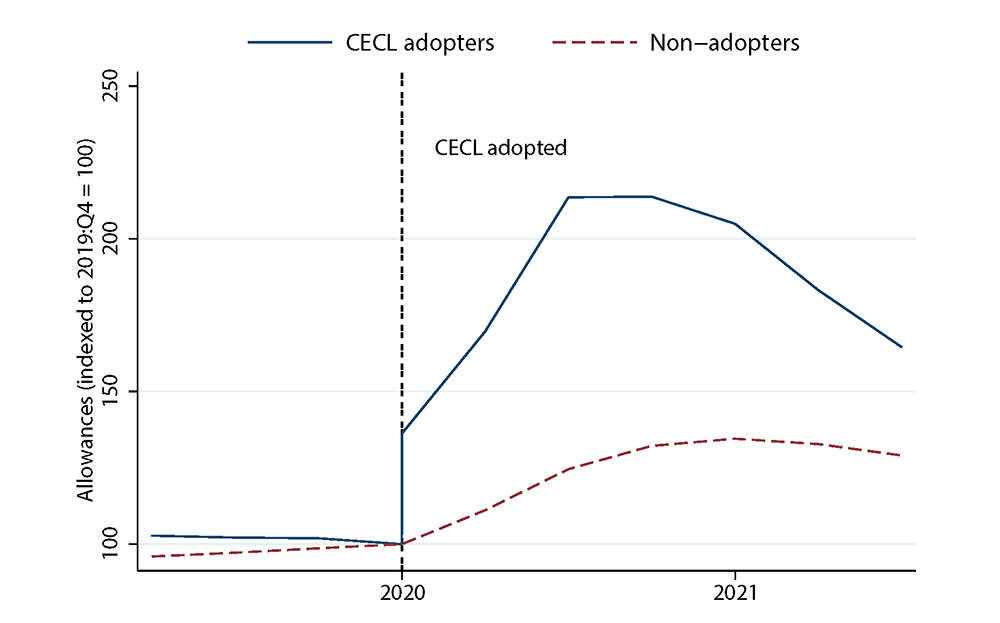
Note: The adoption of CECL results in a jump in allowances (of CECL adopters) on January 1, 2020.
Sources: FR Y-9C filing firms and Call Report firms with filings not consolidated within included filings.
Table 1 shows that the impact of CECL on allowances varies significantly across loan types, presented in descending order of their share of total allowances in 2019:Q4.7 Column (1) provides the January 1, 2020 adoption impact.8 Consumer loan types that tend to have higher credit losses (e.g., credit cards) or longer maturities (e.g., student loans and auto) had the largest increases in allowances at CECL adoption. Columns (2) and (3) show allowance changes in the first half of 2020, as the economic outlook worsened. Allowances of all loan types increased materially during this period, with CECL adopters' allowances increasing more rapidly than non-adopters in each respective portfolio. Columns (4) and (5) show changes in allowances between June 2020 and June 2021 when the economic outlook steadily improved. During this period, CECL adopters substantially decreased their allowances across portfolios whereas non-adopters' total allowances stayed relatively flat in aggregate. At both adopter and non-adopter banks, decreases in allowances during this period were larger for consumer than wholesale loans. Allowances only continued to increase for the commercial real estate portfolio, which may have been adversely affected by the lingering effects of the pandemic on office occupancy rates.
While there are significant differences between the typical CECL adopter and non-adopter that could account for some of the divergence described above, the pandemic experience suggests that CECL generally increases the responsiveness of provisioning for credit losses to changes in economic outlook.
Table 1: Change in Allowances, by Loan Type
Loan Type | Percentage Change in Allowances Relative to Dec 31, 2019 | ||||
---|---|---|---|---|---|
On Jan 1, 2020 | Jan 1, 2020 - June 30, 2020 | June 30, 2020 – June 30, 2021 | |||
CECL Adopters | CECL Adopters | Non-Adopters | CECL Adopters | Non-Adopters | |
[1] | [2] | [3] | [4] | [5] | |
Cards | 48.4 | 71.3 | 33.6 | -46.7 | -19.5 |
Commercial | 7.5 | 99.5 | 33.3 | -70.9 | -10.1 |
Other Consumer* | 97.1 | 46.5 | 55.6 | -44.2 | -31.0 |
Commercial RE | 33.3 | 55.5 | 29.4 | 11.9 | 28.8 |
Residential RE | 27.6 | 81.9 | 19.9 | -72.1 | 7.1 |
Construction | -13.9 | 75.3 | 24.6 | -28.4 | 19.5 |
Total | 37.4 | 75.9 | 32.3 | -49.3 | -0.8 |
Note: CECL adoption impact estimates by loan type are described in endnote 10. RE stands for real estate.
*Other consumer includes all consumer loans other than mortgage and credit cards, and primarily consists of student, auto, and installment loans.
Sources: FR Y-9C and FFIEC Call Reports.
Estimates of CECL's Capital Impact in the Pandemic
Figure 4 shows the evolution of aggregate common equity tier 1 (CET1) capital ratio for CECL adopters—both including (solid black line) and excluding (dashed blue line) the impact of the transition rule.9 With most firms using the transition rule, the aggregate capital ratio declined by about 50 basis points to 11.3 percent in 2020:Q1, and climbed thereafter, reaching 12.5 percent in 2021:Q2. The transition rule increased the ratio by about 30 to 40 basis points, depending on the quarter.
To assess the impact of CECL, we first estimate what would have happened to CECL adopters' allowances—and therefore their capital—if they had remained on ILM. We use data on non-adopter banks to estimate a linear regression relating allowance-to-loan ratios in each quarter to bank and loan characteristics. Then, we use this model to predict what the allowances of CECL adopters would have been if they had remained on ILM.10 Each dollar change in allowances is converted into a negative 79 cent change in capital under the assumption of a 21 percent corporate tax rate. In our estimate, we also assume that CECL had no effect on the dollar amount of net capital distributions (e.g., dividends and share repurchases).
The black dashed lines in the figure represent a range of estimates for what CECL adopters' aggregate capital ratio would have been under ILM. This range is the middle 95 percent of aggregate capital ratios that result from running this regression model on many random samples of the non-adopters, with the size of the range reflecting uncertainty in how the evolution of allowances relates to banks' characteristics.
Our estimates suggest that the transition rule approximately neutralized the aggregate capital impact of CECL so far, with the aggregate capital of CECL adopters falling within or just below the range of estimated outcomes under ILM. However, as suggested by Table 1, the transition rule may not have neutralized the capital impact of CECL across loan types.
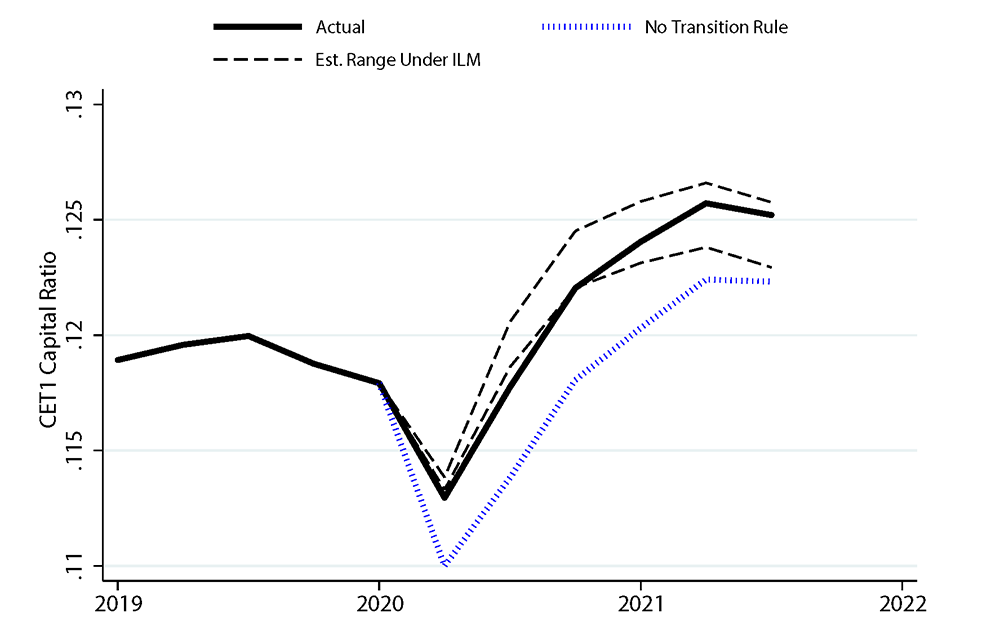
Note: CET1 capital ratio = Common equity tier 1 capital divided by risk-weighted assets.
Sources: FR Y-9C, FFIEC Call Reports, and authors' analysis.
CECL Impact on Lending in the Pandemic
While the pandemic provides evidence that CECL presents less of a "too little, too late" concern, commenters on CECL have suggested that the standard may result in tighter bank credit supply when it is in greatest need.11 The generally larger allowance under CECL reduces capital, causing banks to raise or retain more capital in order to preserve their capital buffers. As capital is considered a more expensive funding source, this potentially increases loan funding costs. Consequently, we might expect CECL adopters to reduce the type of lending with increases in allowances under CECL. However, only the diverse "other consumer" loan type experienced a statistically significant decrease in lending at CECL adopters relative to non-adopters. Moreover, as we discuss further, this difference in growth is difficult to attribute to CECL adoption given the pre-existing difference in other consumer loan growth trends across the two types of banks.
To formally investigate the relationship between CECL's impacts on allowances and loan growth, we follow the well-established difference-in-differences methodology. In this method, the outcomes of treated (CECL adopters indicated by CECLi=1) banks are compared to the outcomes of untreated banks (non-adopters), before and after the treatment occurs (post-CECL adoption indicated by Postt=1). This comparison is made separately for each loan type p for which granular data on allowances are available, using banks i over quarters t (2016:Q1-2021:Q2) using the specification below:
Loan growthp,i,t=βpPostt∗CECLi+3∑n=1γp,nDelinqp,n,i,t+δp,t+θp,i+εp,i,t
Allowance/Loansp,i,t=βpPostt∗CECLi+3∑n=1γp,nDelinqp,n,i,t+δp,t+θp,i+εp,i,t
Note the regression specifications includes additional controls: three delinquency rates (Delinqp,n,i,t), bank-specific fixed effects θp,i, and quarterly fixed effects δp,t.12 Under the assumption that CECL adoption is the only remaining reason for outcomes (allowances or loan growth) to change differently for adopters and non-adopters after Jan 1, 2020, the coefficient βp equals the average effect of CECL on loan growth or additional allowances for loan type p.
This difference-in-differences assumption—and therefore our estimates of CECL impact—is more likely to be valid when the adopter and non-adopter banks are similar. Thus, we remove banks with atypical business models, as well as the largest (mostly CECL adopters) and smallest (mostly non-adopters) from our regression sample.13 We also apply filters to remove immaterial business lines and limit the role of outliers.14 As very few banks in our sample have material card portfolios, we exclude cards from the analysis.15 Finally, we assess the plausibility of the difference-in-differences assumption by testing whether CECL adopters and non-adopters faced similar loan growth trends prior to CECL adoption. The test rejects the hypothesis that CECL adopters and non-adopters' "other consumer" loan growth followed the same trend prior to CECL adoption, which casts doubt on the validity of the comparison of these loans across the two sets of banks.16
The results of our regressions are summarized in Figure 5, with the corresponding regression tables in the appendix (Table A.1 Panel A and B). The values of βp—the average estimated effects of CECL on allowances and loan growth—are represented by the coordinates of the plotted circles, with each circle representing a specific loan type. The y-axis value is the effect on loan growth, and the x-axis value is the effect on allowances. The dashed crosshairs represent statistical 95 percent confidence intervals for these estimates.
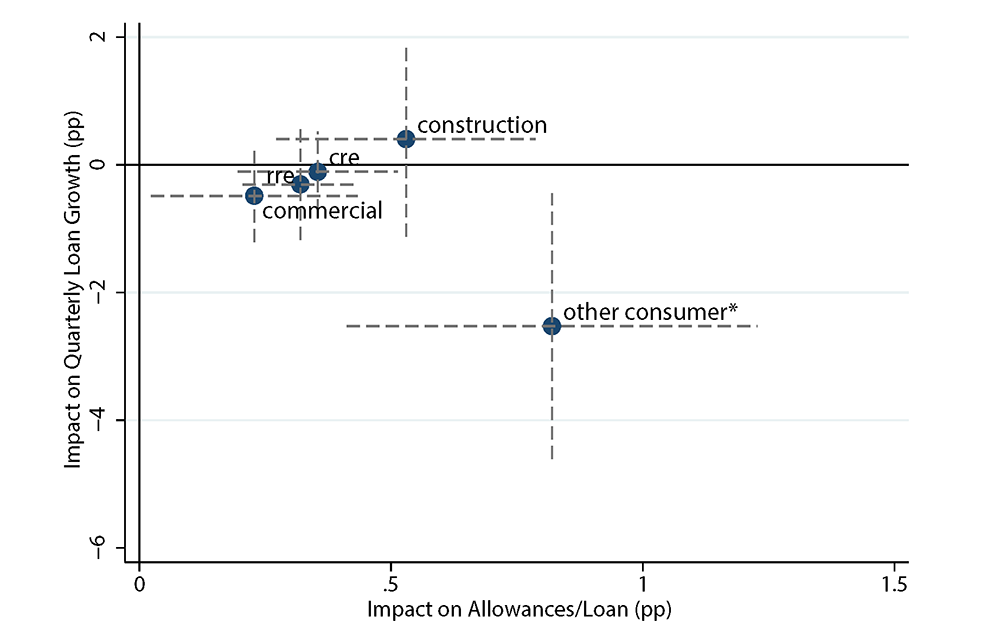
Note: *Other consumer includes all consumer loans other than mortgage and credit cards, and primarily consists of student, auto, and installment loans. Our test rejects the hypothesis of parallel growth trends for other consumer loans prior to CECL adoption (2016-2019). See endnote 16 for further discussion.
Sources: FR Y-9C, FFIEC Call Reports, and authors' analysis.
All five circles are located far enough to the right of the vertical axis that their crosshairs do not cross it—indicating that the average impact of CECL on allowances of each loan type was positive and statistically significant. If CECL's impact on allowances negatively affected loan growth, we should expect the circles to fall below the horizontal axis of Figure 5. Only the "other consumer" loan type shows significantly lower loan growth at CECL adopters following CECL adoption. However, as discussed previously, conclusions based on comparisons of other consumer loans between the two types of banks should be interpreted with caution.
Overall, this analysis does not provide substantial evidence that CECL affected loan growth despite the impact on allowances. One possibility, is that the transition rule sufficiently offset CECL's impact on allowances for each type of loan, suggested by supplemental analysis (Appendix Table A.1 Panel C), and banks factored this benefit into their operational decisions during the unprecedented time of stress.
Conclusion
CECL adopters' loan loss provisioning was noticeably more responsive to the dramatic changes in economic outlook that occurred in the COVID-19 pandemic. In addition, the transition rule performed well in approximately neutralizing the aggregate capital impact of CECL on adopters.
We further explored the impact of CECL on lending. On this point, we interpret the limited evidence with caution. CECL has the potential to reduce lending cyclicality when it acts as a precautionary measure, resulting in banks building allowances as credit risks gradually build in the late stages of the credit cycle. In contrast, the sharp change in the economic outlook during the COVID-19 experience was unlike any other credit cycle in modern times—occurring without and independent of any buildup of credit risk. As such, it is difficult to determine the cyclicality characteristics of CECL. In addition, the sizeable fiscal and monetary policy responses during the pandemic also make it hard to generalize these results to a more standard credit cycle. Regardless, our analysis provides little evidence of an association between CECL's impact on allowances and loan growth across different loan types.
Appendix
Table A.1 presents the results of the regressions corresponding to the equations in the "CECL Impact on Lending" section. Panel A covers the regressions used to estimate CECL's impact on allowances, and Panel B covers regressions of CECL's impact on lending. The values of βp are reported in the first row. Each column corresponds to the results obtained for a specific loan type. In addition to the results discussed above, the controls for delinquency rates show that higher delinquency rates are generally associated with lower lending growth and higher allowances.
In Panel C, we test whether CECL has had an impact on allowances after accounting for the impact of an offset to the capital impact of the allowances. Specifically, as the transition rule adds a capital offset to neutralize 25 percent of (post-adoption) allowance increases while using CECL, we adjust allowances of transition rule users by multiplying the allowance-to-loan ratio after CECL adoption by 0.75. Panel C shows that none of the estimates of impact to adjusted allowances are statistically significant at a ten percent level.
Table A.1 - Regression Estimates of the Impact of CECL on Allowances and Loan Growth, by Loan Type
Panel A: Loan growth
Commercial | Other Consumer | Commercial RE | Residential RE | Construction | |
---|---|---|---|---|---|
VARIABLES | (1) | (2) | (3) | (4) | (5) |
CECL Adopter X Post-2019 | -0.49 | -2.53** | -0.1 | -0.31 | 0.4 |
(0.37) | (1.06) | (0.32) | (0.44) | (0.78) | |
30-Day Delinquency Rate (pp) | -0.38 | -1.38** | -0.23 | -0.91*** | -0.43 |
(0.39) | (0.6) | (0.31) | (0.3) | (0.27) | |
90-Day Delinquency Rate (pp) | -2.57*** | -3.15*** | 0.16 | 0.74*** | -1.1 |
(0.82) | (1.11) | (0.55) | (0.14) | (1.28) | |
Non-Accrual Rate (pp) | -0.84*** | -2.94*** | -1.14*** | -0.66*** | -0.62*** |
(0.2) | (0.79) | (0.17) | (0.27) | (0.22) | |
Fixed Effects | Quarter, Bank | ||||
Observations | 5,325 | 4,554 | 5,359 | 5,344 | 5,078 |
R2 | 0.088 | 0.026 | 0.035 | 0.039 | 0.02 |
Panel B: Allowance/Loan Ratio (pp)
Commercial | Other Consumer | Commercial RE | Residential RE | Construction | |
---|---|---|---|---|---|
VARIABLES | (1) | (2) | (3) | (4) | (5) |
CECL Adopter X Post-2019 | 0.23** | 0.82*** | 0.35*** | 0.32*** | 0.53*** |
(0.1) | (0.17) | (0.08) | (0.06) | (0.13) | |
30-Day Delinquency Rate (pp) | 0.06 | 0.16** | 0.07* | -0.02 | 0 |
(0.05) | (0.07) | (0.04) | (0.02) | (0.02) | |
90-Day Delinquency Rate (pp) | 0.28** | -0.41 | -0.14 | -0.02 | 0.37 |
(0.12) | (0.31) | (0.08) | (0.02) | (0.32) | |
Non-Accrual Rate (pp) | 0.35*** | 0.50*** | 0.07** | 0.08* | -0.01 |
(0.07) | (0.18) | (0.03) | (0.05) | (0.06) | |
Fixed Effects | Quarter, Bank | ||||
Observations | 5,327 | 4,554 | 5,359 | 5,344 | 5,078 |
R2 | 0.169 | 0.096 | 0.122 | 0.086 | 0.048 |
Panel C: Allowance/Loan Ratio – Adjusted for Transition Rule (pp)
Commercial | Other Consumer | Commercial RE | Residential RE | Construction | |
---|---|---|---|---|---|
VARIABLES | (1) | (2) | (3) | (4) | (5) |
CECL Adopter X Post-2019 | -0.16 | 0.27 | 0.02 | 0.08 | 0.12 |
(0.1) | (0.17) | (0.08) | (0.05) | (0.12) | |
30-Day Delinquency Rate (pp) | 0.05 | 0.18*** | 0.06* | -0.01 | -0.01 |
(0.05) | (0.06) | (0.03) | (0.02) | (0.02) | |
90-Day Delinquency Rate (pp) | 0.31** | -0.39 | -0.11 | -0.02 | 0.44 |
(0.13) | (0.27) | (0.07) | (0.01) | (0.35) | |
Non-Accrual Rate (pp) | 0.35*** | 0.46*** | 0.07** | 0.08* | -0.02 |
(0.07) | (0.17) | (0.03) | (0.05) | (0.06) | |
Fixed Effects | Quarter, Bank | ||||
Observations | 5,327 | 4,554 | 5,359 | 5,344 | 5,078 |
R2 | 0.16 | 0.078 | 0.078 | 0.066 | 0.038 |
1. All errors and omissions are our own responsibility. The views expressed in this note are solely the responsibility of the authors and should not be interpreted as reflecting the views of the Board of Governors of the Federal Reserve System or of anyone else associated with the Federal Reserve System. We thank Aaron Garner for able research assistance. Return to text
2. Accounting Standards Update (ASU) 2016-13, ASC Topic 326, "Financial Instruments – Credit Losses." Entities that meet the definition of an U.S. Securities and Exchange (SEC) filer, excluding smaller reporting companies, as defined by the SEC, were required to adopt CECL on January 1, 2020. All other entities are required to adopt CECL on January 1, 2023. Return to text
3. Net charge-offs are charge-offs minus recoveries. The change in allowance equals provision minus charge-off; in other words, positive (negative) provision increases (decreases) allowance and charge-off decreases allowance. Return to text
4. For example, see Baker, Malcolm, and Jeffrey Wurgler. 2015. "Do Strict Capital Requirements Raise the Cost of Capital? Bank Regulation, Capital Structure, and the Low-Risk Anomaly." American Economic Review, 105 (5): 315-20 and Cline, William, "Testing the Modigliani-Miller Theorem of Capital Structure Irrelevance for Banks" (April 2015). Peterson Institute for International Economics Working Paper No. 15-8, Available at SSRN: https://ssrn.com/abstract=2594025 or http://dx.doi.org/10.2139/ssrn.2594025 Return to text
5. Firms adopting CECL on January 1, 2020 held 78 percent of banking system loans as of 2019:Q4, while firms that adopted CECL between January 2, 2020 and June 30, 2021 held only 2 percent of loans as of 2019:Q4. We exclude these late CECL adopters from our analyses. Return to text
6. 85 FR 61577, 9/30/2020 "Regulatory Capital Rule: Revised Transition of the Current Expected Credit Losses Methodology for Allowances." The capital transition rule provides banking organizations that adopt CECL during calendar year 2020 with the option to delay an estimate of CECL's impact on regulatory capital by two years, followed by a three-year transitional period to phase out the aggregate amount of the capital benefit provided during the initial two-year delay. Taken together, these measures offer electing firms a transition period of up to five years. Return to text
7. In 2019:Q4, aggregate allowances were composed of allowances for the following loan types: cards (35%), commercial (29%), other consumer (12%), commercial real estate (12%), residential real estate (8%), and construction (3%). Return to text
8. The adoption impact of CECL is not available at the loan type level in regulatory reports. To estimate the adoption impact by loan type we run a least-squares regression of firm-level adoption impact on 2020:Q1 changes in firm-level allowances by loan type, using 1/(total loans) as a regression weight. Our initial estimate of the loan type impact equals the regression coefficient on the given loan type multiplied by the change in loan type allowances in 2020:Q1. Our reported estimate scales these initial estimates so that the total adoption impact across loan types equals the known aggregate adoption impact. Return to text
9. Of the 178 CECL adopters, 144 (81 percent, representing 96 percent of adopters' total allowances on 2019:Q4) elected to make use of the capital transition rule. Banks opting out of the transition rule were generally either small or had smaller increases—or even decreases—in allowances under CECL. Return to text
10. We aggregate banks at the topholder level, then estimate our regression equation below across all non-adopter banks i with at least $1 billion in loans (as of 2019:Q4), separately in each quarter t after 2019:Q4. Allowance/Loani,t=αt+βtDelinqit+δtChar2019:Q4i+γtAllowance/Loan2019:Q4i+εit. Controls include Delinqit, current bank delinquency characteristics (30 day delinquency rate, 90 day delinquency rate, non-accrual rate—all winsorized at the 99th percentile), Char2019:Q4i, bank characteristics as of 2019:Q4 (average loan interest rate, the share of total loans composed of (i) construction, (ii) commercial real estate, (iii) residential real estate, (iv) commercial or (v) consumer loans, and log total assets winsorized at the 99th percentile of non-adopters size and the allowance-to-loan ratio). Our estimate of what allowances would have been for adopters—if they had remained on ILM—is the fitted value from this regression, multiplied by their actual loans. Return to text
11. E.g., see Covas, Francisco and William Nelson. 2018. "Current Expected Credit Loss: Lessons from 007-2009"(July 2018). Bank Policy Institute Staff Working Paper 2018-1, Available at: https://bpi.com/wp-content/uploads/2018/07/CECL_WP-2.pdf Return to text
12. Delinquency rates are measured at the bank and loan type level in each quarter t, and include 30 day, 90 day and non-accrual rates. We winsorize the rates at the 99th percentile. The bank fixed effects θp,i control for time-invariant explanations specific to the bank i and loan type p. The quarterly fixed effects δp,t control for time-specific explanations applicable to all banks in loan type p, such as general demand for the loan type at t. Return to text
13. First, we remove banks that are likely to behave differently: monoline banks (one loan type exceeds 70 percent of total loans on average) and banks with average loan-to-asset ratios below 25 percent. Next, we remove firms with total assets above $100 billion in 2019:Q4. Then, we draw a lower size threshold (of about $2.3 billion in 2019:Q4 total assets) such that exactly half of the banks in our sample are non-adopters. Return to text
14. Specifically, we remove loan portfolios smaller than $10 million at the end of the previous quarter. We also winsorize loan growth at the 1st and 99th percentiles. Return to text
15. Card lending is particularly concentrated, with just 14 (28) banks accounting for 95 (99) percent of outstanding card balances in 2019:Q4. Return to text
16. Specifically, we run a specification which adds interactions between CECLi and each pre-CECL quarter (2016:Q1-2019:Q4), and then run a Wald test on the hypothesis that the coefficient on these interactions are equal, i.e., that CECL adopters and non-adopters followed parallel growth trends for this period. The p-value for this test statistic for each loan type is: commercial (0.162), other consumer (0.003), commercial RE (0.338), residential RE (0.224) and construction (0.323). Return to text
Loudis, Bert , Sasha Pechenik, Ben Ranish, Cindy M. Vojtech, and Helen Xu (2021). "New Accounting Framework Faces Its First Test: CECL During the Pandemic," FEDS Notes. Washington: Board of Governors of the Federal Reserve System, December 03, 2021, https://doi.org/10.17016/2380-7172.3025.
Disclaimer: FEDS Notes are articles in which Board staff offer their own views and present analysis on a range of topics in economics and finance. These articles are shorter and less technically oriented than FEDS Working Papers and IFDP papers.