FEDS Notes
October 23, 2020
What Drove Recent Trends in Corporate Bonds and Loans Usage?1
Jacob Bochner, Min Wei, and Jie Yang
1. Introduction
U.S. nonfinancial business debt increased substantially in recent years in both absolute and relative terms and is now near its record high.2 Figure 1 shows that most of this increase was due to significant growth in investment-grade (IG) corporate bonds and institutional leveraged loans, while high-yield (HY) corporate bonds and C&I loans largely remained steady.3 This change in debt composition may have been driven by one or both of the following factors: First, firms with existing access to debt markets could have substituted from one type of debt to another. Second, new or improved market access to debt for previously financially constrained firms may have led to growth in certain areas of debt.
In this note, we use firm-level data to shed light on these trends by examining what factors have influenced firms' usage of bond and loan financing and how the composition of bond and loan financing has changed since 2004.4 Overall, we find that the recent growth in IG bonds largely reflects an expansion in the larger, IG-rated segment of firms and their increased usage of bonds. At the same time, HY or unrated, smaller firms have taken the opportunity to issue more floating rate products, as investor demand for such products soared amid low interest rates. The increased loan usage by these firms, together with a larger share of HY firms, contributed to the growth in leveraged loans.
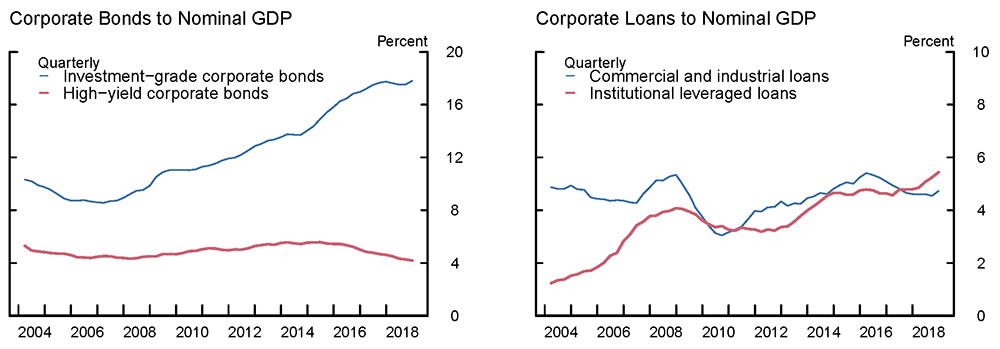
Left Panel - Sources: For nominal GDP, Bureau of Economic Analysis, National Income and Product Accounts; for investment−grade and high−yield corporate bonds, FISD.
Right Panel - Sources: For nominal GDP, Bureau of Economic Analysis, National Income and Product Accounts; for institutional leveraged loans, S&P LCD; for commercial and industrial loans, Federal Reserve Board, Statistical Release Z.1, Financial Accounts of the United States.
2. Sample Description
We begin with an annual, merged sample of firms in Standard & Poor's (S&P) Compustat and Capital IQ databases between 2004 and 2018. We focus on U.S. nonfinancial firms with public information and therefore exclude financial firms, public sector entities, public utilities, subsidiaries, private firms, and firms not incorporated in the United States. We further remove firms with total assets less than $5 million, with less than three years of data, and with apparently erroneous data.5 To minimize discrepancies between Compustat and Capital IQ, we also remove companies whose difference between total debt in the former and the sum across all debt types in the latter is greater than five percent of their total debt. Finally, we exclude firms with missing data on key characteristics including total assets, total debt, cash, book-to-market ratio, EBITDA, capital expenditures, and R&D investment. This gives us a total of 34,896 observations spanning 5,240 unique firms from 2004 to 2018.
Table 1: Sample Tabulation by Capital Structure, Credit Rating, and Size
Capital Structure | S&P Issuer Long-term Credit Rating | Total Asset Size Terciles | |||||
---|---|---|---|---|---|---|---|
Total (1) | IG (2) | HY (3) | Unrated (4) | Large (5) | Medium (6) | Small (7) | |
Zero-Debt | 8910 | 30 | 55 | 8,825 | 591 | 3,259 | 5,060 |
(26%) | (0%) | (1%) | (99%) | (7%) | (37%) | (57%) | |
Loans Only | 7132 | 98 | 508 | 6,526 | 1,012 | 3,206 | 2,914 |
(20%) | (1%) | (7%) | (92%) | (14%) | (45%) | (41%) | |
Bonds Only | 5,323 | 1,384 | 953 | 2,986 | 2,654 | 1,524 | 1,145 |
(15%) | (26%) | (18%) | (56%) | (50%) | (29%) | (22%) | |
Mixed Debt | 13,531 | 2,461 | 4,129 | 6,941 | 7,380 | 3,642 | 2,509 |
(39%) | (18%) | (31%) | (51%) | (55%) | (27%) | (19%) |
Note: IG stands for investment-grade and HY stands for high-yield. Percentages in Column (1) are calculated across capital structure types, while percentages in Columns (2) through (4) and (5) through (7) are calculated across credit rating or size categories, respectively, within each capital structure type.
Source: S&P Compustat and S&P Capital IQ.
We split our sample into four categories based on capital structure: those with 1) no debt, 2) only loans outstanding, 3) only bonds outstanding, and 4) both bonds and loans outstanding. Column (1) of Table 1 tabulates the sample across these four capital structure categories. Within each capital structure category, we further show the distributions across three credit rating groups based on S&P's issuer-level long-term credit rating (Columns 2 through 4) and across three equal-sized bins based on total assets (Columns 5 through 7). Not surprisingly, almost all zero-debt firms are unrated and the majority are classified as small firms, while almost half of the mixed-debt firms have ratings and the majority are classified as large firms. Indeed, the correlation between firm size and credit rating quality is about 0.64 in our sample. Meanwhile, loans-only firms follow similar size and rating distributions as zero-debt firms, while bonds-only firms follow similar distributions as mixed debt firms. Although the majority of firms in both the loans- and bonds-only categories are unrated, among those who do have ratings, a larger proportion of bonds-only (loans-only) firms are rated IG (HY).
Table 2: Mean Firm Characteristics by Capital Structure, Credit Rating, and Size
Firm Type | Assets ($billion) | Market Capitalization ($billion) | B-t-M of Equity | Debt/ Assets |
Cash/ Assets |
Earnings/ Assets |
Firms | |
---|---|---|---|---|---|---|---|---|
(1) | Zero-Debt | 0.5 | 1.41 | 0.54 | 0 | 0.44 | -0.01 | 2074 |
(2) | Loans-Only | 0.72 | 1.08 | 0.64 | 0.19 | 0.16 | 0.06 | 2174 |
(3) | Bonds-Only | 8.89 | 11.62 | 0.5 | 0.23 | 0.22 | 0.07 | 1713 |
(4) | Mixed-Debt | 6.24 | 6.04 | 0.58 | 0.33 | 0.09 | 0.1 | 2790 |
Investment-Grade Firms | ||||||||
(5) | Loans Only | 6.51 | 14.01 | 0.38 | 0.16 | 0.09 | 0.18 | 37 |
(6) | Bonds Only | 28.3 | 38.46 | 0.37 | 0.25 | 0.12 | 0.16 | 292 |
(7) | Mixed Debt | 21.14 | 24.2 | 0.41 | 0.28 | 0.09 | 0.15 | 407 |
High-Yield Firms | ||||||||
(8) | Loans Only | 1.76 | 1.78 | 0.47 | 0.36 | 0.09 | 0.13 | 216 |
(9) | Bonds Only | 4.21 | 2.77 | 0.59 | 0.33 | 0.16 | 0.11 | 352 |
(10) | Mixed Debt | 5.17 | 2.94 | 0.59 | 0.42 | 0.07 | 0.11 | 880 |
Unrated Firms | ||||||||
(11) | Loans Only | 0.55 | 0.84 | 0.66 | 0.18 | 0.16 | 0.06 | 2006 |
(12) | Bonds Only | 1.38 | 2.01 | 0.54 | 0.19 | 0.29 | 0 | 1184 |
(13) | Mixed Debt | 1.59 | 1.44 | 0.63 | 0.3 | 0.11 | 0.07 | 1871 |
Firms in Largest Size Tercile | ||||||||
(14) | Loans Only | 3.11 | 5.02 | 0.53 | 0.21 | 0.12 | 0.14 | 376 |
(15) | Bonds Only | 17.52 | 22.84 | 0.44 | 0.25 | 0.16 | 0.14 | 683 |
(16) | Mixed Debt | 11.08 | 10.72 | 0.52 | 0.35 | 0.08 | 0.13 | 1280 |
Firms in Middle Size Tercile | ||||||||
(17) | Loans Only | 0.55 | 0.73 | 0.62 | 0.2 | 0.12 | 0.11 | 1045 |
(18) | Bonds Only | 0.57 | 0.84 | 0.55 | 0.24 | 0.26 | 0.08 | 602 |
(19) | Mixed Debt | 0.69 | 0.69 | 0.63 | 0.33 | 0.09 | 0.11 | 1067 |
Firms in Smallest Size Tercile | ||||||||
(20) | Loans Only | 0.07 | 0.1 | 0.71 | 0.18 | 0.21 | -0.02 | 1055 |
(21) | Bonds Only | 0.05 | 0.1 | 0.59 | 0.16 | 0.31 | -0.13 | 577 |
(22) | Mixed Debt | 0.06 | 0.06 | 0.68 | 0.29 | 0.13 | 0 | 866 |
Note: Sample extends from FY 2004 through FY 2018. Ratings are from S&P and at the issuer level. Capital structure groups are generated based on outstanding values in each fiscal year.
Sources: Compustat; Capital IQ; S&P.
Table 2 provides the mean firm characteristics across our capital structure categories, for all firms in Rows (1) to (4), firms within a certain credit rating group in Rows (5) through (13), and firms within a size tercile in Rows (14) through (22). Generally, firms with bonds-only or mixed debt are larger and more levered than firms with zero or loans-only debt.6 In addition, mixed-debt firms appear to be less financially constrained potentially due to their easier access to external financing as they hold less cash while realizing higher earnings.7 Among IG firms, the differences between mixed debt and other debt (bonds-only or loans-only) firms are smaller. However, among unrated firms, loans-only firms appear to be financially healthier than bonds-only firms as they have higher earnings to assets. Similarly, the differences are smaller across debt categories among firms in the largest tercile, but within the middle and smaller groups, loans-only firms appear healthier than bonds-only firms.8
3. Determinants of Corporate Bonds and Loans Usage
First, we explore the determinants of bonds and loans usage at the firm level using regression analysis. Following extant literature, we focus on the impact of credit rating and firm size on those debt structure choices.9
In addition, the choice between bond and loan financing may also be affected by the interest rate outlook. Market commentaries suggest that when rates are expected to rise, investors appear to prefer floating rate products, such as leveraged loans, as opposed to fixed rate products, such as corporate bonds.10 Banks may also increase their lending in such an environment, as expectations of higher rates typically occur against a strengthening economic outlook in our sample. However, when rates are expected to rise, firms may have the opposite incentive and prefer the lower borrowing cost of the fixed rate corporate bonds than the potentially higher costs of floating rate loans. Given that firms' sizes and ratings affect their debt structure choices and debt market access, we expect that the impact of interest rate outlook on debt structure outcomes would also depend on the size and rating of the firm. In our analysis, we measure the interest rate outlook using the spread between the 2- and 10-year Treasury yields, adjusted for term premiums.11 Absent term premiums, a steeper expected short rate path would push up longer-term yields more than shorter-term ones, resulting in a wider term spread.
3.1. Shares of Bonds and Loans in Total Debt
Our first regression is a probit model relating the likelihood that a firm is bonds-only or loans-only to the firm's rating and size characteristics as well as the current term spread, controlling for other factors:
(1) P(CSTypei,t=1)=α+β1Has LT Credit Ratingi,t+β2Has IG Credit Ratingi,t+β3Log(Total Assetsi,t)+β4Adjusted Term Spreadt+β5Log(Total Assetsi,t)×Adjusted Term Spreadt+Other Controlsi,t+Year Fixed Effectst+εi,t
Where CSType is either Bonds-Only or Loans-Only for firm i in year t, HasLTCreditRating is an indicator variable that takes the value of 1 if the firm has a long-term S&P issuer credit rating and 0 otherwise, HasIGCreditRating is an indicator variable that takes the value of 1 if the firm has an IG long-term S&P issuer credit rating and 0 otherwise, and Log(Total Assets) is our measure for firm size. As mentioned above, we measure interest rate outlook using the 2- to 10-year term spread, adjusted for the term premium component (Adjusted Term Spread).12
Our second regression is an OLS model relating a firm's shares of bonds or loans in total debt, as well as its bonds-to-loans ratio, to firm size, credit rating, and the interest rate outlook:
(2) CSVari,t=α+β1Has LT Credit Ratingi,t+β2Has IG Credit Ratingi,t+β3Log(Total Assetsi,t)+β4Adjusted Term Spreadt+β5Log(Total Assetsi,t)×Adjusted Term Spreadt+Other Controlsi,t+Year Fixed Effectst+εi,t
where CSVar is the bond or loan share or the bonds-to-loans ratio for firm i in year t. In both specifications (1) and (2), we include typical firm characteristic controls such as book equity-to-market equity ratio, earnings scaled by total assets, capital expenditure scaled by total assets, research and development expense scaled by total assets, long-term debt scaled by total assets, and short-term debt scaled by total assets. We also include fiscal year fixed effects to capture any time trends.
Table 3 provides the results. Columns (1) and (2) show the results from probit regressions in Eq. (1) and Columns (3) through (5) show the results from OLS regressions in Eq. (2). Consistent with Table 1, firms with credit ratings, in particular those with IG ratings, are more likely to be bonds-only (Column 1) or use a larger share of bonds in their debt structure (Column 3), as indicated from the positive and significant coefficients on the Has LT Credit Rating and Has IG Credit Rating variables, respectively. Whereas, having a HY rating or no rating at all leads firms to more likely be loans-only (Column 2) or use a higher proportion of loans in their debt structure (Column 4). Similarly, larger (smaller) firms are more likely to be bonds- (loans-) only or use a larger proportion of bonds (loans), as indicated by the statistically significant coefficient on Log(Total Assets). These results are consistent with, but additional to, the results on firm size as larger firms are more likely to have a rating and be IG rated, while smaller firms are less likely to have a rating and when they do, more likely to get a HY rating.
In general, higher expected future interest rates, as proxied by a wider term spread adjusted for term premiums, is associated with less bonds usage and more loans usage, consistent with the narrative that banks and/or loan investors supply more loans when interest rates are expected to rise. However, as expected, the relationship between interest rate outlook and debt structure depends on firm size. In particular, we find the effect of adjusted term spread becomes smaller for larger firms, as evidenced by the coefficients on the interaction term, Log(Total Assets)×Adjusted Term Spread. In all cases (except Column (2)), the coefficients on the interaction term are significant with opposite signs to the coefficients on Adjusted Term Spread itself. For the median-sized firm in our sample (approximately $500 million), the individual effect of Adjusted Term Spread dominates the effect of the interaction term. However, the effect of the interaction term dominates that of the adjusted term spread alone for firm sizes above approximately $5 billion, approximately the size of our average firm. These results are consistent with the interpretation that larger firms, with more bargaining power and more options in their capital structure, will use more fixed-rate bonds to avoid potentially higher borrowing costs in the future when interest rates are expected to rise. By contrast, smaller firms, though lacking bargaining power and options, may have been able to take advantage of the increased supply of loan financing by banks and/or investors over the same period.
Table 3: Regression Analysis on the Determinants of Debt Structure
(1) Probit | (2) Probit | (3) OLS | (4) OLS | (5) OLS | |
---|---|---|---|---|---|
Bonds-Only | Loans-Only | Total Bonds / Total Debt | Total Loans / Total Debt | Total Bonds / Total Loans | |
HasLTCreditRating | 0.083** | -0.693*** | 0.167*** | -0.143*** | 10.790*** |
(2.257) | (-10.401) | (10.006) | (-8.814) | (3.129) | |
HasIGCreditRating | 0.527*** | -0.524*** | 0.169*** | -0.205*** | 68.955*** |
(9.664) | (-9.020) | (10.993) | (-16.010) | (8.372) | |
Log (Total Assets) | 0.045*** | -0.052*** | 0.030*** | -0.034*** | 2.344 |
(4.030) | (-7.196) | (11.550) | (-13.756) | (1.736) | |
Adjusted Term Spread | -0.195*** | -0.007 | -0.057*** | 0.058*** | -22.306*** |
(-3.840) | (-0.230) | (-5.093) | (4.660) | (-4.789) | |
Log (Total Assets) x Adjusted Term Spread | 0.021*** | -0.008** | 0.007*** | -0.007*** | 2.950*** |
(3.041) | (-2.032) | (3.548) | (-3.493) | (3.205) | |
Constant | -1.634*** | -0.387*** | 0.199*** | 0.740*** | 112.701*** |
(-26.910) | (-8.997) | (8.254) | (26.112) | (14.821) | |
Observations | 34,896 | 34,896 | 25,986 | 25,986 | 25,780 |
Pseudo/Adjusted R-squared | 0.063 | 0.090 | 0.248 | 0.249 | 0.094 |
Controls? | Y | Y | Y | Y | Y |
Year Fixed Effects? | Y | Y | Y | Y | Y |
3.2. Changes in Bonds and Loans Usage Relative to Assets
Having shown that credit rating and size are important determinants of bond and loans usage in a firm's debt structure, we next examine how firms in different ratings or size groups changed the levels and relative shares of bonds and loans over time.
Tables 4 classifies firms within each rating and size category, respectively, into 9 categories – shown as 3-by-3 matrices – based on how they change their bonds-to-assets ratios and their loans-to-assets ratios since the previous year. In each year, firms with at least a one percentage point increase (decrease) in either debt ratio from the previous year is grouped into the "increase" ("decrease") category, while firms with less than a one percentage point change are categorized as "same". When firms increase (decrease) both bonds and loans usage relative to assets, they unambiguously increase (decrease) their leverage positions. However, when firms increase one form of debt while decreasing another form of debt, we classify these actions as substitution behavior.
Table 4: Changes in Bonds-to-Assets and Loans-to-Asset Ratios
Panel A: Across Credit Rating Categories
Make Full ScreenInvestment-Grade Firms | High-Yield Firms | Unrated Firms | |||||||
---|---|---|---|---|---|---|---|---|---|
Change in Loans/Assets |
Change in Loans/Assets |
Change in Loans/Assets |
|||||||
Change in Bonds/Assets |
Decrease (1) |
Same (2) |
Increase (3) |
Decrease (4) |
Same (5) |
Increase (6) |
Decrease (7) |
Same (8) |
Increase (9) |
Decrease (1) |
3.7% De-Levered |
21.2% | 8.0% Substitution |
6.9% De-Levered |
14.1% | 16.4% Substitution |
3.4% De-Levered |
6.1% | 5.6% Substitution |
Same (2) |
6.2% | 22.2% Unchanged |
4.8% | 13.9% | 12.6% Unchanged |
7.3% | 15.7% | 45.3% Unchanged |
13.1% |
Increase (3) |
8.0% Substitution |
21.5% | 4.4% Levered Up |
14.4% Substitution |
8.0% | 6.4% Levered Up |
4.1% Substitution |
3.8% | 2.9% Levered Up |
Panel B: Across Size Categories
Make Full ScreenLarge Firms | Medium Firms | Small Firms | |||||||
---|---|---|---|---|---|---|---|---|---|
Change in Loans/Assets |
Change in Loans/Assets |
Change in Loans/Assets |
|||||||
Change in Bonds/Assets |
Decrease (1) |
Same (2) |
Increase (3) |
Decrease (4) |
Same (5) |
Increase (6) |
Decrease (7) |
Same (8) |
Increase (9) |
Decrease (1) |
4.9% De-Levered |
15.8% | 11.3% Substitution |
3.3% De-Levered |
6.6% | 6.8% Substitution |
3.5% De-Levered |
4.8% | 4.2% Substitution |
Same (2) |
10.9% | 20.9% Unchanged |
7.2% | 18.1% | 40.4% Unchanged |
14.1% | 13.8% | 52.5% Unchanged |
12.6% |
Increase (3) |
10.9% Substitution |
12.8% | 5.3% Levered Up |
4.4% Substitution |
3.6% | 2.8% Levered Up |
2.8% Substitution |
3.1% | 2.8% Levered Up |
Panel A of Table 4 shows that the most frequent change for IG firms is to adjust their bonds usage up or down, while maintaining their loans usage unchanged (Columns 1 through 3). By contrast, substitution – particularly into loans – plays a larger role for HY firms (Columns 4 through 6). Finally, not surprisingly, most unrated firms kept their capital structures little changed across time (Columns 7 through 9). For unrated firms that did change their capital structures, the main course of action appears to be adjusting loans usage, while maintaining their previous bonds (or lack thereof) positions. These results are consistent with the interpretation that IG firms have more bargaining power that allows them to access the cheaper bond market, while unrated firms appear to have gained better access to debt markets via loans. Meanwhile, HY firms appear to have access to both markets and engage more in substitution based on what is optimal. Panel B of Table 4 shows that substitution plays a larger role for large firms than medium or small firms, while the latter two types of firms engage in capital structure adjustments mainly through changes in their loans usage.
Next, we explicitly test the relations observed above using probit regression analysis by running Equation (1) using the following left-hand side variables:
- An indicator variable that takes the value of 1 (0) if a firm experienced an increase (decrease) in its bonds to assets ratio,
- An indicator variable that takes the value of 1 (0) if a firm experienced an increase (decrease) in loans to assets ratio,
- An indicator variable that takes the value 1 (0) if a firm unambiguously levered up (down),
- An indicator variable that takes the value 1 (0) if a firm substituted one form of debt for another (unambiguously levered up or down), and
- An indicator variable that takes the value 1 (0) if a firm substituted from loans into bonds (substituted from bonds into loans).
Table 5: Probit Regression Analysis on Changes in Debt Structure
(1) Probit | (2) Probit | (3) Probit | (4) Probit | (5) Probit | |
---|---|---|---|---|---|
Increase in Bonds/Assets (1) vs. Decrease in Bonds/Assets (0) | Increase in Loans/Assets (1) vs. Decrease in Loans/Assets (0) | Levered Up (1) vs. Levered Down (0) | Substitution (1) vs. Levering Up or Down (0) | Substitute into Bonds (1) vs. Substitute into Loans (0) | |
HasLTCreditRating | -0.054 | 0.085** | 0.061 | 0.084 | -0.059 |
(-1.223) | (2.535) | (0.598) | (1.394) | (-0.976) | |
HasIGCreditRating | 0.104* | -0.003 | -0.094 | -0.069 | 0.071 |
(1.647) | (-0.050) | (-0.688) | (-0.904) | (0.830) | |
Log (Total Assets) | 0.056*** | 0.029* | 0.091*** | 0.013 | 0.051* |
(2.898) | (1.917) | (3.539) | (0.861) | (1.943) | |
Adjusted Term Spread | -0.011 | 0.100 | -0.032 | -0.344*** | -0.050 |
(-0.057) | (0.778) | (-0.090) | (-3.262) | (-0.308) | |
Log (Total Assets) x Adjusted Term Spread |
0.010 | -0.021 | 0.007 | 0.021** | 0.037** |
(0.685) | (-1.213) | (0.444) | (2.045) | (2.202) | |
Constant | -0.337 | 0.027 | 0.245 | 0.105 | -0.597** |
(-1.526) | (0.200) | (0.702) | (0.794) | (-2.520) | |
Observations | 10,122 | 12,645 | 2,042 | 5,734 | 3,692 |
Pseudo/Adjusted R-squared | 0.0340 | 0.0360 | 0.119 | 0.0189 | 0.0559 |
Controls? | Y | Y | Y | Y | Y |
Year Fixed Effects? | Y | Y | Y | Y | Y |
As shown in Column (1) of Table 5, having a larger firm size or an IG rating increases the likelihood of increasing bonds. In Column (2), having a rating, but not IG rating (i.e., having a HY rating) increases the likelihood of increasing loans. In Column (3), not surprisingly, larger firms are more likely to lever up (versus de-levering). In Column (4), the adjusted term spread becomes significant, with a higher spread decreasing the likelihood of substitution relative to that of adjusting leverage up or down. This would be consistent with firms gaining access to the loan market and levering up during these periods amid increased investor demand for floating rate products. However, the interaction with size is also significant, suggesting that larger firms are more likely to substitute when rates are expected to rise, as they have the flexibility of accessing either the bond or loans market as discussed previously. Finally, in Column (5), larger firms are more likely to substitute into bonds versus loans, particularly during periods of higher expected future rates when they have relatively more bargaining power to issue lower cost fixed-rate bonds.
4. What Drove the Recent Trends in Corporate Bonds and Loans Usage?
Having examined the determinants of bonds and loans usage at the firm-level in the previous section, we now explore our central question: how can the growth trends in different types of debt, as noted in the beginning of this note, be explained by changes in the composition of firms along rating and size dimensions and in firms' financing behaviors.
Figure 2 shows the time series evolutions of the average firm's bonds to assets and loans to asset ratios within each credit rating category in Panel A and within each size tercile in Panel B. As shown in Panel A, IG firms on average use more bonds and less loans relative to assets than the other two firm types. HY firms appear to be the most leveraged among the three groups, using as much bonds as IG firms and more loans than others relative to their assets. Finally, unrated firms appear to use the lowest leverage, with the least bond usage and with loans usage between those of IG firms and HY firms. Over our sample, IG firms increased their bond usage and HY firms increased their loans usage, relative to assets, while the other debt usages remained relatively steady. As shown in Panel B, likewise to IG firms, the largest firms rely much more on bond financing, while medium and smaller firms rely more on loan financing. Since 2004, the largest firms have increased both their bond and loan financing, the mid-sized firms have increased loan financing while maintaining their bond share, and the smallest firms have reduced their bond financing while keeping the loan financing unchanged.
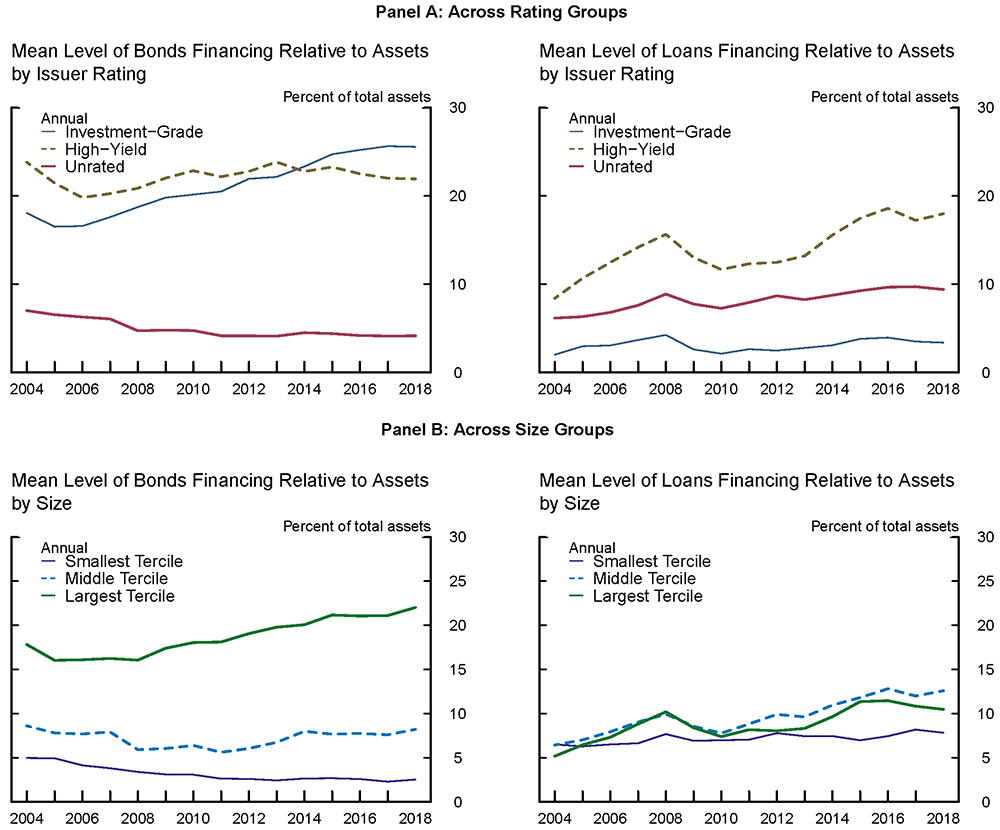
Panel A: Across Rating Groups
Left Panel: Mean Level of Bonds Financing Relative to Assets by Issuer Rating.
Note: Data show the mean level of bonds outstanding as a percent of total assets for the average firm. Sources: Compustat; Capital IQ; S&P.
Right Panel: Mean Level of Loans Financing Relative to Assets by Issuer Rating.
Note: Data show the mean level of loans outstanding as a percent of total assets for the average firm. Sources: Compustat; Capital IQ; S&P.
Panel B: Across Size Groups
Left Panel: Mean Level of Bonds Financing Relative to Assets by Size.
Note: Data show the mean level of bonds outstanding as a percent of total assets for the average firm. Sources: Compustat; Capital IQ.
Right Panel: Mean Level of Bonds Financing Relative to Assets by Size.
Note: Data show the mean level of loans outstanding as a percent of total assets for the average firm. Sources: Compustat; Capital IQ.
Finally, Figure 3 zooms in on 2004 and 2018, the first and last year of our sample, and asks how the compositional changes, both across and within the rating (left column) and size (right column) groups, have contributed to the change in the aggregate capital structure between those two years.
Panel A (top left) shows changes in the rating composition: over time, more firms become rated, as the proportion of unrated firms shrinks and the proportions of IG, and especially HY, firms increase. As rated firms tend to issue more bonds and less loans relative to assets than unrated firms, this shift by itself would likely have led to higher bond usage over our sample. Panel B (middle left) shows how the capital structure has shifted for an average firm within each rating group. As already seen from Figure 2, IG firms, the two rightmost bars, on average increased their bond-to-asset ratios while kept the loan-to-asset ratios relatively unchanged between 2004 and 2018. In contrast, both HY (two middle bars) and unrated firms (two rightmost bars) significantly reduced their bond usage while at the same time increasing their loan usage. Together, these observations suggest that much of the recent increase in loan usage could be attributed to both a shift from unrated firms into HY and an increased usage of loans by HY and unrated firms, the latter of which possibly due to the rapid growth of the leveraged loans market. Meanwhile, the shift from unrated firms into IG firms and the increased usage of bonds by IG firms likely contributed to the increase in IG bonds outstanding. Reflecting these changes, Panel C (bottom left) shows significant shifts from unrated to IG in the share of bonds and from unrated to HY in the share of loans between 2004 and 2018.
The panels on the right-hand side of Figure 3 conduct a similar analysis for the three size groups. In this case, the composition of the size terciles, shown in Panel D (top right), is fixed by construction.13 However, within each size tercile, we observe distinct trends that are consistent with what we see for the rating groups. In particular, in Panel E (middle right), the largest firms (two leftmost bars) increased their bond usage, though at a slower pace than IG firms, as well as their loan usage. By contrast, both the mid-sized firms (two middle bars) and the smallest firms (two rightmost bars) saw large expansions of loan usage and the smallest firms reduced their bond usage. These observations suggest that the increase in loan usage seen in our sample might be a consequence of small to mid-sized firms gaining or expanding access to the loan market while at the same reducing their reliance on the bond market. Despite this trend, as shown in Panel F (bottom right), the largest firms continue to dominate not only the bond but also the loan market and their loan market share barely diminishes over our sample, reflecting the much larger issue size by those firms.
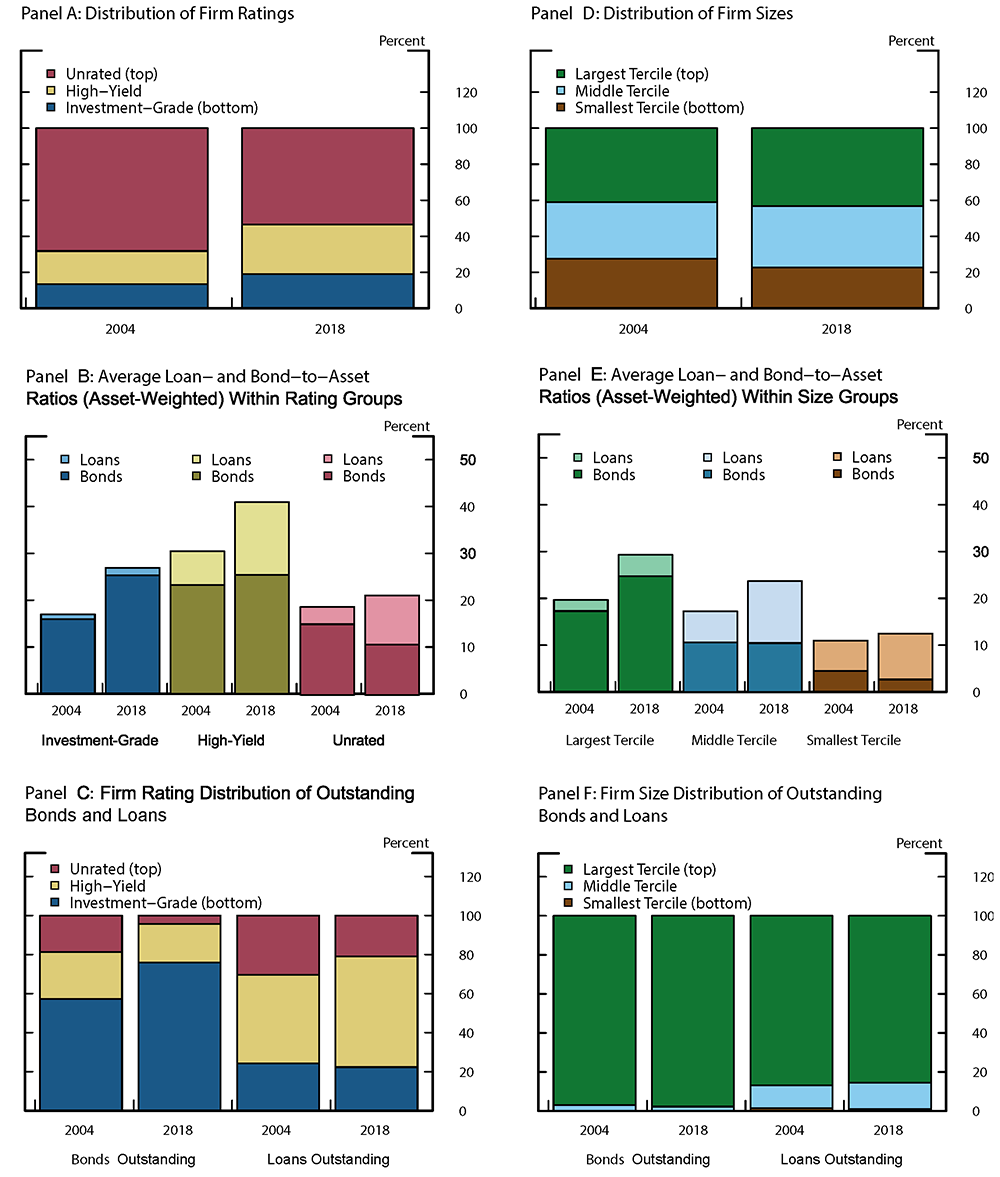
References
Almeida, H., M. Campello, and M.S. Weisbach, 2004, The Cash Flow Sensitivity of Cash, Journal of Finance, 59, 1777-1804.
Colla, P., Ippolito, F., and Li, K., 2013, Debt Specialization, Journal of Finance, 68, 2117-2141.
Frank, M.Z. and V.K. Goyal, 2009, Capital Structure Decisions: Which Factors Are Reliably Important?, Financial Management, 38, 1-37.
Goldfarb, S. 2019, Fed Expectations Trigger Record Run of Outflows in Leveraged Loans, the Wall Street Journal, https://www.wsj.com/articles/fed-expectations-trigger-record-run-of-outflows-in-leveraged-loans-11562601551.
Gürkaynak, R. S., Sack, B., and Wright, J. H., 2007, The U.S. Treasury yield curve: 1961 to the present. Journal of monetary Economics, 54(8), 2291–2304.
Hadlock, C.J. and J.R. Pierce, 2010, New Evidence on Measuring Financial Constraints: Moving Beyond the KZ Index, Review of Financial Studies, 23, 1909-1940.
Kim, D. H. and A. Orphanides, 2012, Term Structure Estimation with Survey Data on Interest Rate Forecasts, Journal of Financial and Quantitative Analysis, 47(1), pp. 241-272.
Kim, D. H. and J. H. Wright, 2005, An Arbitrage-Free Three-Factor Term Structure Model and the Recent Behavior of Long-Term Yields and Distant-Horizon Forward Rates, Federal Reserve Board Finance and Economics Discussion Series 2005-33.
Opler, T., L. Pinkowitz, R. Stulz, R. Williamson, 1999, The Determinants and Implications of Corporate Cash Holdings, Journal of Financial Economics, 52, 3-46.
Whited, T. and G. Wu, 1996, Financial Constraints Risk, Review of Financial Studies, 19, 531-559.
Standard & Poor's. Compustat Xpressfeed North America, via Wharton Research Data Services.
Standard & Poor's. CapitalIQ/Capital Structure, via Wharton Research Data Services.
Van Binsbergen, J.H., J.R. Graham, and J. Yang, 2010, The Cost of Debt, Journal of Finance, 60, 2089-2136.
1. We thank Marco Cagetti, Eric Engstrom, Giovanni Favara, Felicia Ionescu, Camelia Minoiu, Kate Proctor, and Rebecca Zarutskie for helpful discussions and suggestions. All remaining errors are our own. Return to text
2. Federal Reserve Board (2019). "Financial Stability Report (PDF)". Washington: Board of Governors of the Federal Reserve System, November 2019. Return to text
3. Data obtained from Y-14Q reports to the Federal Reserve since 2011 suggest that C&I loans in large bank holding companies are about equally distributed between IG and HY ratings. Rating categories are constructed by mapping internal ratings provided by the banks to standardized external ratings using bank documentation provided to the Federal Reserve. Return to text
4. This analysis focuses on the expansion in debt before the recent COVID-19 episode. Return to text
5. These include firms with one-year asset growth of 1000 percent or -90 percent, total debt or cash greater than total assets, negative cash, and total bonds or total loans greater than total debt. Return to text
6. These findings are similar to Colla, Ippolito, and Li (2013), who document that larger, more levered, or rated firms tend to employ multiple types of debt, while smaller, unrated firms tend to specialize in one type of debt. Return to text
7. See , e.g., Opler, Pinkowitz, Stulz, and Williamson (1999) and Almeida, Campello, and Weisbach (2004). These papers show that firms with greater access to capital markets, i.e., less financially constrained, tend to hold less cash to non-cash assets and exhibit less sensitivity in their cash holdings to earnings. Return to text
8. These results may seem counterintuitive, but likely reflect a sample selection bias: only the healthiest unrated or smaller firms are able to gain access to external capital markets, typically starting with the loan market. As those firms mature and gain access to the bond market, their financial health is likely to deteriorate to levels more in line with that of an average firm in their rating or size group. Return to text
9. For example, Almeida and Campello (2004) use having a credit rating as well as firm size as proxies for a firm's access to external capital markets. Meanwhile, Whited and Wu (2006), Frank and Goyal (2009), Hadlock and Pierce (2010), and van Binsbergen, Graham, and Yang (2010) find that, among other factors, firm size is one of the most reliably significant factors in the firm's capital structure decision, degree of being financially constrained, and cost of debt. Return to text
10. For example, in a Wall Street Journal article, Goldfarb (2019) cited interest rate outlooks as factors driving both the large inflows into leveraged loan funds in 2018 and the large outflows in early 2019. Return to text
11. In particular, we subtract the difference between the 2- and 10-year term premiums from the term spread to isolate the expectations component. We take the term premium estimates from Kim and Orphanides (2012) and Kim and Wright (2005), available at https://www.federalreserve.gov/data/three-factor-nominal-term-structure-model.htm. Treasury yields are constructed using the method outlined in Gürkaynak, Sack and Wright (2007) and downloaded from https://www.federalreserve.gov/data/nominal-yield-curve.htm. Return to text
12. We do not interact interest rate outlook with credit rating variables in our specifications due to multicollinearity between credit rating and firm size. Return to text
13. Recall that by construction, we create equal-sized terciles based on asset size. Return to text
Bochner, Jacob, Min Wei, and Jie Yang (2020). "What Drove Recent Trends in Corporate Bonds and Loans Usage?," FEDS Notes. Washington: Board of Governors of the Federal Reserve System, October 23, 2020, https://doi.org/10.17016/2380-7172.2789.
Disclaimer: FEDS Notes are articles in which Board staff offer their own views and present analysis on a range of topics in economics and finance. These articles are shorter and less technically oriented than FEDS Working Papers and IFDP papers.