FEDS Notes
July 19, 2024
Global Implications of Brighter U.S. Productivity Prospects
Danilo Cascaldi-Garcia and Hyunseung Oh1
Investment related to artificial intelligence (AI) is booming in the U.S. compared to other countries, as shown in figure 1. Moreover, new business registrations have surged in the U.S. since the pandemic, unlike in the euro area where new business registrations remained flat (de Soyres et al. 2024). This surge in new business formation has been driven disproportionately by the high-tech industry, which has contributed sizably to past productivity fluctuations (Decker and Haltiwanger 2024; Fernald 2015). Do these developments suggest the U.S. is on the verge of a higher path for productivity growth, and if so, how might that productivity gain spill over to other countries?
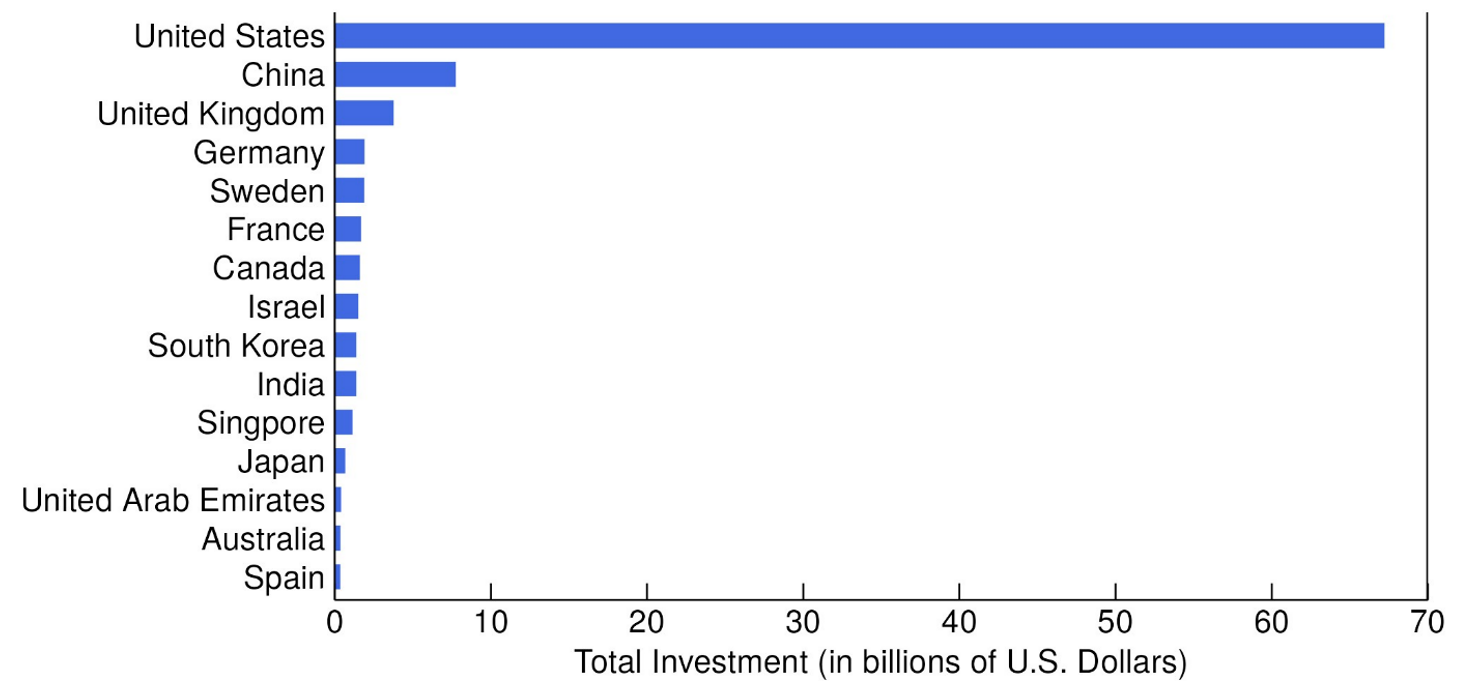
Note: Data taken from Maslej et al. (2024).
Source: Institute for Human-Centered AI, Stanford University.
In this note, we study the global implications of an expected surge in future U.S. productivity. Our methodology is based on the insight that, because new technologies take time to develop and diffuse, some future productivity gains are anticipated well ahead of time and are reflected in firms' investment and stock prices. We use a vector autoregression model (VAR) that includes productivity, investment, and stock prices to recover a shock—known in the economics literature as a news shock—that best anticipates the future evolution of U.S. total factor productivity (TFP) over history. We use the news shock to investigate (1) how current expectations for future U.S. productivity compare to the IT boom and bust period during the 1990s, (2) how a shock to future U.S. productivity affects the economy, and (3) how such a shock spills over to other advanced economies.
The Model
Our methodology allows us to decompose movements in TFP into surprise TFP shocks and news shocks, that is, shocks to expected future TFP. As innovation diffuses slowly and new technological developments announced today may only impact TFP with a lag, news shocks better capture the effects of innovation and technological development on TFP. Indeed, economic agents that are aware that the economy will be more productive in the future should react to such information today. Consistent with this intuition, the empirical literature has shown that a positive news shock induces an increase in GDP, investment, and to a lesser extent, consumption, even before any productivity increase is realized (e.g., Beaudry and Portier, 2006; Barsky and Sims, 2011; Cascaldi-Garcia and Galvao, 2021).
We employ an identification strategy similar to Barsky and Sims (2011). The method takes the utilization-adjusted TFP measure calculated by Fernald (2014) as a proxy for the productivity level of the economy, and defines the two productivity shocks (surprise and news) as the ones that maximize unexplained movements in TFP. Surprise TFP shocks explain all the contemporaneous movements in TFP, and news shocks explain the largest share of such movements in the long-run, conditional on being orthogonal to surprise shocks. Specifically, the news shock can be identified within a VAR by solving the optimization problem:
$$$$ \gamma_{2}^{news} = \underset{\gamma}{argmax} ( \frac{\sum_{h=0}^{H} IRF_{h}^{TFP} \gamma \gamma {'} IRF_{h}^{TFP} {'}} {\sum_{h=0}^{H} V_{h}^{TFP}} ) $$$$
subject to $$ \gamma (1,1) = 0 $$ and $$ \gamma \gamma {'} = 1 $$, where $$ IRF_{h}^{TFP} $$ is the impulse response at each $$h$$ step ahead of a TFP shock identified recursively, $$ V_{h}^{TFP} $$ is the total TFP variance at each $$h$$ step ahead, $$ \gamma $$ is an identification vector, and $$ \gamma_{2}^{news} $$ is the resulting optimal vector that represents the structural mapping between the VAR shock and the news shock. In words, the solution to this problem yields a news shock that best explains movements in TFP $$H$$ periods in the future, conditional on attributing all contemporaneous TFP movements to a surprise shock.2
The model is estimated with Bayesian methods and 4 lags. Following Cascaldi-Garcia (2022), we also include Pandemic Priors in the VAR for the 4 quarters of 2020 to downplay the strong variations during the onset of the pandemic and prevent it from influencing the parameter estimates.
Data
Our VAR includes 13 quarterly variables, with 8 variables from the U.S. and 5 variables expressed as a differential between the U.S. and an aggregate of other advanced economies. We define the other advanced economies as the purchasing power parity GDP-weighted aggregate of the rest of the G-7 countries, namely, Canada, France, Germany, Italy, Japan, and the U.K., which we refer to as G-6 hereafter. As our interest is in the international spillover channel, the information set is enriched by the exchange rate. Therefore, our sample starts from the first quarter of 1973, when the Bretton Woods system of fixed exchange rates was effectively abolished and many countries allowed their exchange rates to float. The sample ends at the fourth quarter of 2023.
The U.S. variables included are the utilization-adjusted TFP series from Fernald (2014), the S&P 500 index, real GDP, real personal consumption expenditures, real private fixed investment, labor productivity measured as output per hour worked, the GDP deflator, and the financial uncertainty index constructed by Ludvigson, Ma, and Ng (2021). The variables that are expressed as a differential between the U.S. and the G-6 are real GDP, labor productivity, consumer price index, the nominal exchange rate, and the short-term interest rate. We take logs for each variable except for the interest rate.
Estimation Results
We employ the methodology described above to recover our news shock, or a shock to expected U.S. TFP growth over the next 10 years. Figure 2 presents its trailing six-quarter moving average. Consistent with the IT boom-bust period, the shock was notably positive during the mid to late-1990s, shaded in yellow, and negative in the early 2000s. Recently, we have seen its re-emergence, consistent with AI-related expectations, although the shock is not yet near the peak of the 1990s boom when 10-year-ahead TFP expectations were repeatedly revised up. As such, our estimated shocks are consistent with the narrative that the U.S. might be on the verge of higher productivity in the future, even though the empirical evidence up to 2023 is not yet close to the 1990s boom.
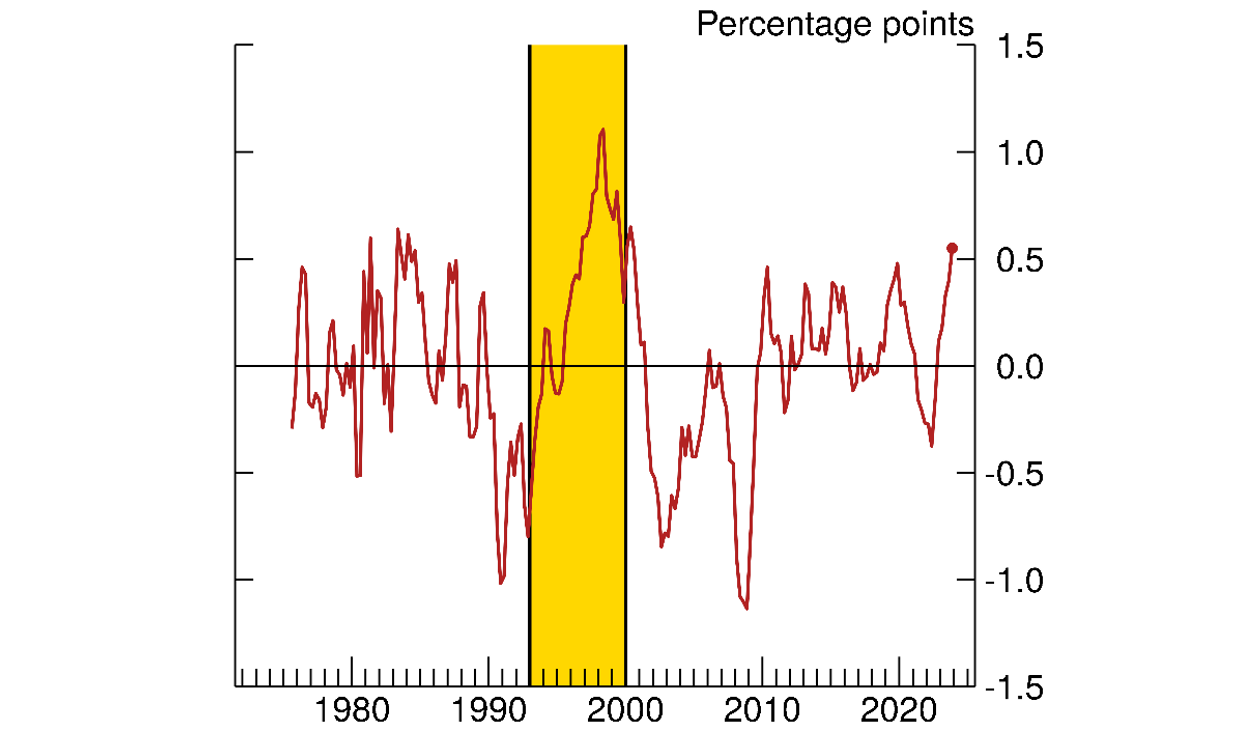
Note: The trailing six-quarter moving average of the shock is plotted. A 1 percentage point increase of the shock implies a 1 percent increase in the 10-year ahead TFP growth prospects. Shaded area covers 1993 to 1999.
Source: National sources via Haver Analytics; FRB staff calculations.
Assuming expected future U.S. productivity does continue to surge, what might the international implications be? We consider a virtuous scenario in which TFP 10 years out is suddenly expected to be an extra 1 percent higher in the U.S., similar to how much TFP prospects were revised up each quarter at the peak of the 1990s boom (figure 3). Following the shock, TFP does not increase right away (with the zero-impact response by design), but rather gradually, with almost 70 percent of the expected TFP gains having been realized after 5 years.
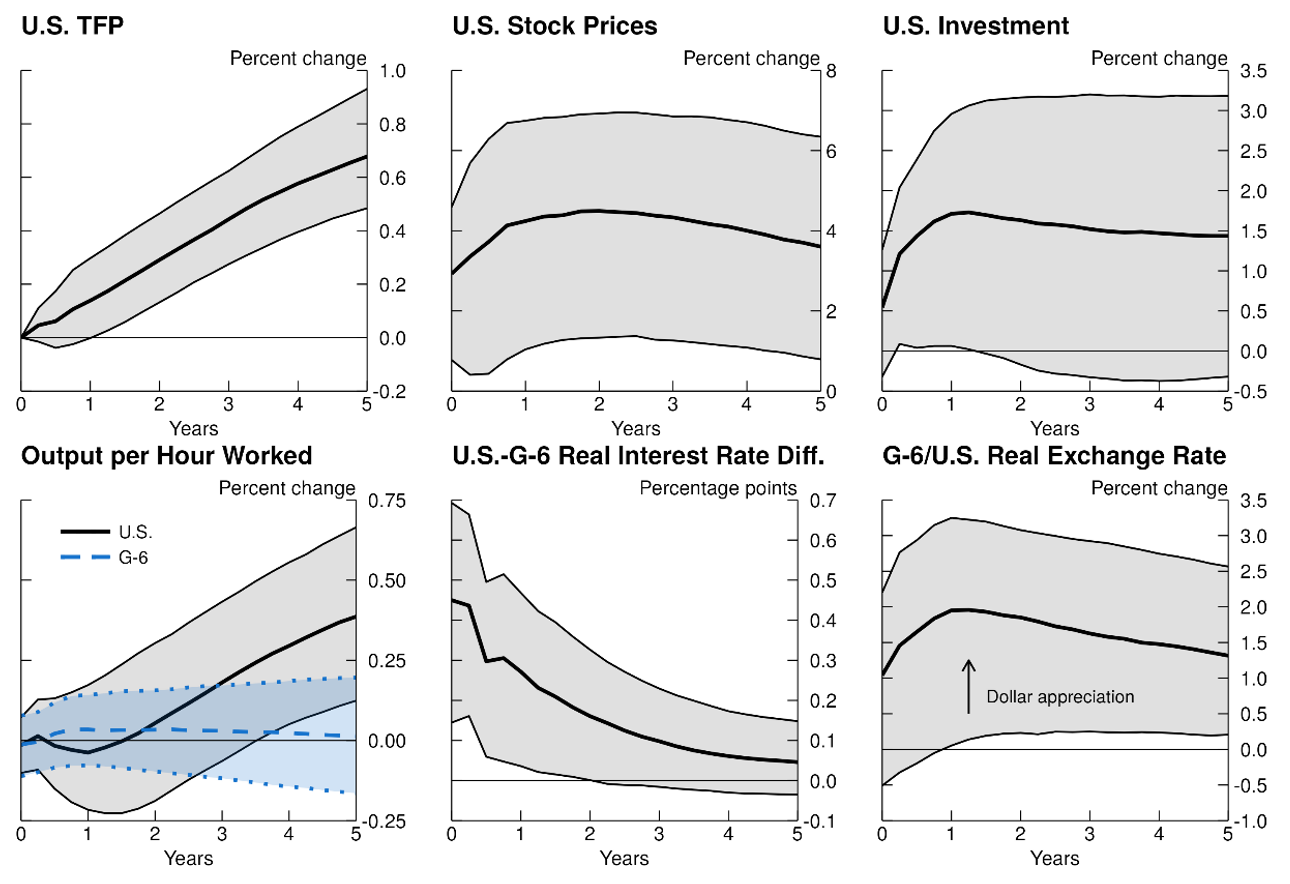
Note: Data are impulse responses years after the initial shock. Shaded areas indicate one standard-deviation bands of uncertainty.
Source: Haver Analytics; FRB staff calculations.
As shown in the top-right panel, given historical relationships, the productivity of workers, defined as output per hour worked, is essentially unaffected over time in other advanced economies, even as the productivity of U.S. workers steadily tracks the TFP gains. Given better U.S. productivity prospects, stock prices, investment, and GDP all increase in the U.S. ahead of the rise in potential output, pushing up the real interest rate. However, no such demand pressures emerge in the other advanced economies, leading to a persistent increase in the real interest rate differential between the U.S. and G-6. In turn, the wider interest rate differential contributes to an appreciation of the dollar.3
Discussion and Conclusion
The above analysis suggests that the recent AI boom is consistent with some optimism about future growth in U.S. productivity. However, it is still too early to say that the U.S. will indeed experience a boost in productivity like in the late 1990s and early 2000s following the IT boom period. In terms of the international implications, improved prospects for U.S. productivity could fuel some divergence in the natural rate of interest between the U.S. and G-6 and an appreciation of the dollar, as history suggests that productivity abroad is not expected to improve in response to positive news shocks to future U.S. productivity.4 The lack of productivity spillovers may sound counterintuitive as technology and ideas should have no borders. One possible explanation for this finding is that the U.S. stands out in terms of business dynamism and financing conditions for new businesses, which may position it better to develop and adopt new technologies.
The future is uncertain, forecasting is hard, and this is just one of the many possible scenarios. But, all told, history suggests that brighter prospects for U.S. productivity could amplify the growth divergence between the U.S. and other advanced economies and lead to the further strengthening of the dollar.
References
Barsky, R. B. and E. R. Sims (2011), "News Shocks and Business Cycles." Journal of Monetary Economics, 58 (3), 273–289.
Beaudry, P. and F. Portier (2006), "Stock Prices, News, and Economic Fluctuations." American Economic Review, 96 (4), 1293–1307.
Cascaldi-Garcia, D. (2022), "Pandemic Priors." International Finance Discussion Paper, 1352.
Cascaldi-Garcia, D. and B. Galvão (2021), "News and Uncertainty Shocks." Journal of Money, Credit and Banking, 53 (4), 779–811.
Chahrour, R., V. Cormun, P. De Leo, P. Guerron-Quintana, and R. Valchev (2024), "Exchange Rate Disconnect Revisited." NBER Working Paper, 32596.
Decker, R. and J. Haltiwanger (2024), "High Tech Business Entry in the Pandemic Era." FEDS Notes, Washington: Board of Governors of the Federal Reserve System, April 19, 2024.
de Soyres, F., J. Garcia-Cabo Herrero, N. Goernemann, S. Jeon, G. Lofstrom, and D. Moore (2024), "Why is the U.S. GDP Recovering Faster than Other Advanced Economies?" FEDS Notes, Washington: Board of Governors of the Federal Reserve System, May 17, 2024.
Fernald, J. (2014), "A Quarterly, Utilization-Adjusted Series on Total Factor Productivity." Federal Reserve Bank of San Francisco Working Paper, 2012-19.
Fernald, J. (2015), "Productivity and Potential Output Before, During, and After the Great Recession." NBER Macroeconomics Annual, 29 (1), 1–51.
Francis, N., M. T. Owyang, J. E. Roush, and R. DiCecio (2014), "A Flexible Finite-Horizon Alternative to Long-Run Restrictions with an Application to Technology Shock." Review of Economics and Statistics, 96, 638–647.
Klein, M. and L. Linnemann (2021), "Real Exchange Rate and International Spillover Effects of U.S. Technology Shocks." Journal of International Economics, 103414.
Ludvigson, S. C., Ma, S., and Ng, S. (2021), "Uncertainty and Business Cycles: Exogenous Impulse or Endogenous Response?" American Economic Journal: Macroeconomics, 13 (4), 369–410.
Maslej, N., L. Fattorini, R. Perrault, V. Parli, A. Reuel, E. Brynjolfsson, J. Etchemendy, K. Ligett, T. Lyons, J. Manyika, J. C. Niebles, Y. Shoham, R. Wald, and J. Clark (2024), "The AI Index 2024 Annual Report." AI Index Steering Committee, Institute for Human-Centered AI, Stanford University, Stanford, CA, April 2024.
Miyamoto, W., T. L. Nguyen, and H. Oh (forthcoming), "In Search of Dominant Drivers of the Real Exchange Rate." Review of Economics and Statistics.
Nam, D. and J. Wang (2015), "The Effects of Surprise and Anticipated Technology Changes on International Relative Prices and Trade." Journal of International Economics, 97, 162–177.
1. We thank our colleagues at the Federal Reserve Board for comments on an earlier draft. Grace Lofstrom and Chris Machol provided excellent research assistance. The views expressed in this note are solely the responsibility of the authors and should not be interpreted as reflecting the views of the Board of Governors of the Federal Reserve System or of anyone else associated with the Federal Reserve System. Return to text
2. We follow Barsky and Sims (2011) by setting H=40 quarters and find the shock that maximizes the share of the forecast-error variance of TFP at horizon h=H as in Francis, Owyang, and DiCecio (2014). Return to text
3. The exchange rate effect of TFP news shocks in the U.S. is also highlighted in the literature by, e.g., Nam and Wang (2015), Klein and Linnemann (2021), and Chahrour et al. (2024). Return to text
4. It is important to note that our analysis is conditional on TFP news shocks; the realized exchange rate response could be vastly different because exchange rate dynamics are sensitive to other shocks less connected to economic fundamentals, as shown by Miyamoto, Nguyen, and Oh (forthcoming). Return to text
Cascaldi-Garcia, Danilo, and Hyunseung Oh (2024). "Global Implications of Brighter U.S. Productivity Prospects," FEDS Notes. Washington: Board of Governors of the Federal Reserve System, July 19, 2024, https://doi.org/10.17016/2380-7172.3559.
Disclaimer: FEDS Notes are articles in which Board staff offer their own views and present analysis on a range of topics in economics and finance. These articles are shorter and less technically oriented than FEDS Working Papers and IFDP papers.