FEDS Notes
April 19, 2024
High tech business entry in the pandemic era
Ryan Decker and John Haltiwanger1
The COVID-19 pandemic and its aftermath have featured a surge in business entry (Decker and Haltiwanger 2024). A natural question is whether the elevated entry seen in recent years will have positive implications for aggregate productivity growth given the historically important role of business entry for productivity dynamics (Decker et al. 2014, Alon et al. 2018). A closely related question is whether the recent entry surge featured strong high tech entry, since high tech industries have been important for historical productivity fluctuations (Fernald 2015). Indeed, the high tech industries that contributed most to the strong productivity growth of the mid-1990s through the early 2000s had seen an earlier surge in firm entry during the early 1990s (Foster et al. 2021).
In this note, we show that high tech industries made a large, disproportionate contribution to the aggregate net establishment entry surge after 2019. Net entry in high tech industries was higher than in non-tech industries during the post-2019 period, even relative to the time before the pandemic; this can be seen in figure 1. Tech industries also made strong contributions to aggregate job creation, including through jobs created by establishment entry. The new facts we document suggest that tech investments did play an important role in recent business entry dynamics. High tech industries making large contributions to aggregate net establishment entry include computer systems design, technical consulting services, software publishers, data processing and hosting, and scientific research and development services, among others.
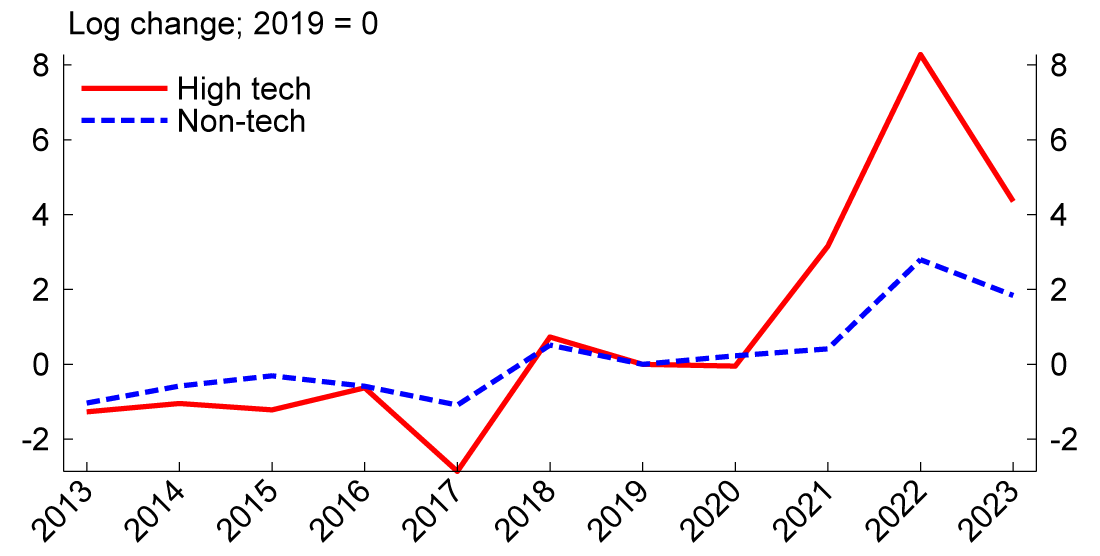
Note: Annual (log) gains versus 2019; average gains weighted by industry average 2013-2019 establishments. High tech defined as in Business Dynamics Statistics.
Source: QCEW.
Our main exercises focus on net entry of employer establishments, which reflects both incumbent firm "greenfield" establishments and entrepreneurial startups (new firms or companies).2 A thorough analysis of the relative roles of incumbent versus new firms must await more detailed data. But we find suggestive evidence that, at least through early 2023, high tech establishment births rose more for incumbent firms than for new firms. However, both kinds of firms saw elevated tech births, and timely business application data suggest new firm creation in key tech industries has remained strong through early 2024—pointing to high anticipated new firm entry over the next couple of years. Both kinds of investment can be thought of as an extensive margin of business activity. Such investments, regardless of organizational form, could be important contributors to aggregate productivity growth over time and may provide hints into the dynamics of high tech investments in the pandemic and post-pandemic economy.
Background
In Decker and Haltiwanger (2024) we compiled evidence from multiple public sources to characterize the recent business entry surge along many dimensions.3 Applications for new business tax identifiers in the Census Bureau's Business Formation Statistics (BFS) rose sharply starting in mid-2020, followed by rising entry of actual new employer firms and establishments starting in 2021 with significant related job creation.4 The surge in business entry is consistent with broader pandemic themes, exhibiting geographic restructuring from downtown areas to suburbs and a tight geographic correlation with the surge in worker quits.
In Decker and Haltiwanger (2024) we also highlighted notable industry patterns in the business entry surge. In particular, in broad sector-level tabulations we found that the tech-intensive sectors of information and professional and business services saw particularly elevated business applications and application-based predicted firm births.5 The BFS features a series predicting actual firm births within two years based on detailed business application microdata, and these two tech-intensive sectors account for nearly one-quarter of the pandemic rise in predicted births. In Decker and Haltiwanger (2024) we also noted elevated actual employer firm and establishment births in these sectors through early 2022 in BLS data, which has continued into early 2023.
But in that paper we did not explore high tech patterns in detail. In the present note we use data on net establishment entry at narrower levels of industry detail to more precisely track high tech industries.
Net establishment entry is similar to other measures of business entry in that it jumped in the pandemic. This can be seen in figure 1, which reports the annual net growth (in logs) of establishment counts from the Bureau of Labor Statistics (BLS) Quarterly Census of Employment and Wages (QCEW), indexed to 2019. Net establishment entry was particularly strong in 2022 and 2023. Over the broader pandemic period (2020-2023), the aggregate (private) establishment count rose by 1.7 million, an increase of nearly 18 percent.
Data
To study high tech entry during recent years, we require a high level of industry detail and reasonably timely data. For these reasons, in our main exercises we use public tabulations from the QCEW, which allow us to study net establishment entry in narrow industries as recently as 2023. Importantly, QCEW does not allow us to distinguish new establishments of existing firms from entrepreneurial startups (we will return to this topic below using different data). Additionally, by only measuring net entry (i.e., the net change in industry establishment counts), we conflate gross entry and exit.6
We study annual net entry (from first quarter to first quarter) during 2013-2023.7 We study a balanced panel of 261 4-digit NAICS industries that account for roughly 90 percent of 2019 QCEW establishments and employment.8
Following the literature, we categorize industries by high tech intensity based on the prevalence of technology-related occupations in the industry.9 We provide results using three different definitions of high tech.
- The first high tech definition, "BDS-HT," follows the high tech categorization used in the Census Bureau's Business Dynamics Statistics High Tech data (which is based in large part on Goldschlag and Miranda 2020). This is our most restrictive categorization, with only 11 4-digit NAICS industries counting as high tech.10
- The second high tech definition, which is less restrictive covering 47 industries, follows Hecker (2005) and has been used in some past research on high tech business dynamics (e.g., Decker et al. 2016 and Haltiwanger, Hathaway, and Miranda 2014).11
- For the third high tech measure, we use actual industry-level STEM employment shares constructed by Goldschlag and Miranda (2020). We use a constant industry-level share that is the average for the industry during 2013-2019 (i.e., the pre-pandemic period). In some analyses we use STEM employment share as a continuous variable, while in others we define "high tech" industries as those at or above the median STEM employment share—a much broader definition than the other concepts we use. This variable is missing for 16 of our 261 industries.
In additional exercises studying the relative roles of incumbent versus new firms, we use data from the BLS Business Employment Dynamics (BED) and from the BFS as we will describe below.
High tech establishment entry was strong in the pandemic era
Table 1 reports the top 20 industries ranked by net establishment gains from 2019 through 2023, measured as log differences (multiplied by 100). That is, we subtract the log establishment count in 2019 from the log establishment count in 2023 such that the results can be interpreted like a growth rate. The three rightmost columns of the table indicate whether an industry is a high tech industry based on our three definitions described above.
Table 1: Ranking 4-digit industries by establishment entry (logs)
Rank | Industry | Growth (logs x 100) | High tech | |||
---|---|---|---|---|---|---|
BDS-HT | Hecker | Top 50% STEM | ||||
1 | 5112 | Software Publishers | 97 | X | X | X |
2 | 5182 | Data Processing, Hosting, and Related Services | 71 | X | X | X |
3 | 3346 | Manufacturing and Reproducing Magnetic and Optical Media | 67 | X | X | |
4 | 3122 | Tobacco Manufacturing | 65 | X | ||
5 | 5251 | Insurance and Employee Benefit Funds | 56 | X | ||
6 | 5417 | Scientific Research and Development Services | 53 | X | X | X |
7 | 5259 | Other Investment Pools and Funds | 53 | X | ||
8 | 3159 | Apparel Accessories and Other Apparel Manufacturing | 47 | |||
9 | 6114 | Business Schools and Computer and Management Training | 46 | X | ||
10 | 3254 | Pharmaceutical and Medicine Manufacturing | 44 | X | X | |
11 | 6117 | Educational Support Services | 43 | X | ||
12 | 5418 | Advertising, Public Relations, and Related Services | 37 | X | ||
13 | 3361 | Motor Vehicle Manufacturing | 37 | X | ||
14 | 5611 | Office Administrative Services | 36 | X | ||
15 | 5415 | Computer Systems Design and Related Services | 36 | X | X | X |
16 | 5416 | Management, Scientific, and Technical Consulting Services | 35 | X | X | |
17 | 2122 | Metal Ore Mining | 35 | X | ||
18 | 8133 | Social Advocacy Organizations | 34 | X | ||
19 | 6221 | General Medical and Surgical Hospitals | 33 | |||
20 | 6219 | Other Ambulatory Health Care Services | 32 |
Note: Top 20 4-digit NAICS industries by log establishment change (x100), 2019 to 2023. Last three columns indicate tech status by, respectively, BDS-HT definitions, Hecker (2005), or STEM share of employment based on STEM measures from Goldschlag & Miranda (2020).
Source: BLS Quarterly Census of Employment and Wages.
Table 1 shows a striking prevalence of high tech industries among the fastest-growing industries of recent years. Out of 11 industries categorized as high tech under the narrow BDS-HT definition, 4 are among the top 20 industries for pandemic era establishment gains. The far right column shows that almost all of the top 20 industries have above-median STEM employment shares.
BDS-HT tech industries' average establishment growth from 2019 through 2023 was nearly three times as high as non-tech industries (37 versus 13 log points); Hecker (2005) tech industries' growth was almost twice as high as non-tech industries (21 versus 13 log points); and industries meeting the broadest tech definition (above-median STEM employment share) grew 50 percent faster than others (18 versus 11 log points). This tech growth advantage tends to be even larger if we weight mean growth in tech versus non-tech using 2019 establishment counts; that is, the tech overperformance is not driven by small, unimportant industries.
Table 1, by measuring establishment gains with log differences, provides insight into within-industry growth in recent years. Table 2 provides simple establishment count changes in levels, affording a view into which industries help account for the aggregate increase in establishments. Computer systems design and related services contributed a stunning 120,000 establishments to the overall change. Tech-related consulting services made a similar contribution.
Table 2: Ranking 4-digit industries by establishment entry (levels)
Rank | Industry | Change (1000s) | High tech | |||
---|---|---|---|---|---|---|
BDS-HT | Hecker | Top 50% STEM | ||||
1 | 5415 | Computer Systems Design and Related Services | 120 | X | X | X |
2 | 5416 | Management, Scientific, and Technical Consulting Services | 114 | X | X | |
3 | 5310 | Real Estate | 71 | |||
4 | 5112 | Software Publishers | 53 | X | X | X |
5 | 4840 | Truck Transportation | 42 | |||
6 | 7225 | Restaurants and Other Eating Places | 40 | |||
7 | 5613 | Employment Services | 40 | X | ||
8 | 6213 | Offices of Other Health Practitioners | 37 | |||
9 | 5419 | Other Professional, Scientific, and Technical Services | 33 | X | ||
10 | 5230 | Securities, Commodity Contracts, and Other Financial Investments and Related Activities | 33 | X | ||
11 | 2361 | Residential Building Construction | 31 | |||
12 | 5412 | Accounting, Tax Preparation, Bookkeeping, and Payroll Services | 31 | X | ||
13 | 5413 | Architectural, Engineering, and Related Services | 27 | X | X | X |
14 | 5617 | Services to Buildings and Dwellings | 27 | |||
15 | 5182 | Data Processing, Hosting, and Related Services | 26 | X | X | X |
16 | 5418 | Advertising, Public Relations, and Related Services | 24 | X | ||
17 | 5511 | Management of Companies and Enterprises | 24 | X | X | |
18 | 5611 | Office Administrative Services | 23 | X | ||
19 | 5417 | Scientific Research and Development Services | 22 | X | X | X |
20 | 2382 | Building Equipment Contractors | 22 |
Note: Top 20 4-digit NAICS industries by establishment change (1000s), 2019 to 2023. Last three columns indicate tech status by, respectively, BDS-HT definitions, Hecker (2005), or STEM share of employment based on STEM measures from Goldschlag & Miranda (2020).
Source: BLS Quarterly Census of Employment and Wages.
Table 3 reports, for each tech definition, the share of 2019 establishments accounted for by high tech industries and the share of the aggregate establishment increase from 2019 through 2023 that is accounted for by high tech industries. For each definition, tech industries' growth contribution is disproportionate to their overall share of the economy.
Table 3: Tech’s disproportionate contribution to aggregate establishment gains
Tech definition | Share of 2019 establishments (%) | Share of 2019-2023 increase (%) |
---|---|---|
BDS-HT | 7 | 19 |
Hecker (2005) | 14 | 31 |
Top 50% STEM | 39 | 56 |
Note: Top 50% STEM defined using Goldschlag & Miranda (2020) STEM shares. Top 50% STEM row limited to industries with STEM employment data.
Source: BLS Quarterly Census of Employment and Wages.
An alternative way to judge the role of high tech industries in recent aggregate economic growth is to focus on employment. The QCEW does not permit tracking the employment of new establishments specifically, but we can observe industry-level employment changes.12 Similar to the establishment-based results in table 3, we find that high tech industries accounted for a disproportionate share of aggregate employment growth from 2019 through 2023—in fact, the employment shares are very similar to those shown for establishments in table 3. High tech industries by the BDS-HT, Hecker (2005), and top 50% STEM definitions created 1.0 million, 1.6 million, and 2.4 million jobs, respectively, from 2019 through 2023.
High tech versus non-tech industries in the pandemic era versus earlier
We have shown that high tech industries played a disproportionate role in net establishment gains seen since 2019. But tech industries tend to grow faster than others more generally, so the data reported above do not necessarily establish a pickup of high tech growth in the pandemic period relative to other industries. We next conduct a more formal analysis of high tech versus non-tech establishment gains in the pandemic period versus the pre-pandemic period. We do not argue that our exercises will uncover a causal effect of the pandemic on growth; for example, non-pandemic-related developments around artificial intelligence may account for some high tech industry dynamics.
Consider a simple difference-in-differences regression framework:
$$$$ g_{it}=\alpha_i + \delta \cdot I \{t \geq 2020\}+ \beta \cdot Tech_i \cdot I\{t \geq 2020\} + \varepsilon_{it} $$$$
In this specification, $$ g_{it} $$ is annual (log) establishment count change (multiplied by 100) for industry i, $$ \alpha_i $$ is an industry fixed effect, $$ I\{t \geq 2020\} $$ is an indicator variable that turns on for years 2020 and after, and $$ Tech_i $$ indicates whether an industry is high tech or not (in the case of the BDS-HT and Hecker 2005 definitions) or is a continuous variable that is the STEM share of industry employment (average 2013-2019) expressed in standard deviations. We do not need to include a $$ Tech_i $$ main effect since it is constant over time for industries.
This specification has a straightforward difference-in-differences interpretation: $$ \delta $$ gives the simple increase in average annual establishment gains for the pandemic versus pre-pandemic period for non-tech industries (or, in the case of the continuous STEM share regressions, $$ \delta $$ reports the pandemic versus pre-pandemic gains for a hypothetical industry with zero STEM employment). $$ \beta $$ gives the additional growth difference for tech versus non-tech in the pandemic versus before the pandemic (or, in the case of the STEM share tech variable, $$ \beta $$ reports the additional gains associated with a standard deviation increase in industry STEM share).
The results from the regression just described are shown in table 4. Each column represents a separate regression corresponding to each high tech definition. The top panel reports results from simple unweighted regressions. The bottom panel reports weighted regressions using the 2013 through 2019 average industry establishment count as weights.
Table 4: The pandemic high tech growth advantage
(1) BDS-HT | (2) Hecker | (3) STEM share | |
---|---|---|---|
A. Unweighted regressions | |||
I{year≥2020} | 2.0*** | 2.0*** | 1.7*** |
(0.2) | (0.2) | (0.2) | |
Tech * I{year≥2020} | 3.3*** | 1.2** | 0.8*** |
(0.8) | (0.4) | (0.2) | |
Observations | 2871 | 2871 | 2695 |
B. Establishment count-weighted regressions | |||
I{year≥2020} | 1.8*** | 1.6*** | 1.5*** |
(0.2) | (0.2) | (0.2) | |
Tech * I{year≥2020} | 3.1** | 2.9*** | 0.7*** |
(1.1) | (0.7) | (0.2) | |
Observations | 2871 | 2871 | 2695 |
Note: t statistics in parentheses; * p < 0.05, ** p < 0.01, *** p < 0.001
Unit of observation is 4-digit NAICS industry-year. Dependent variable is 100*log annual change in industry establishment count. Column headings specify "Tech" definition. STEM share is industry STEM employment share expressed in standard deviations. Huber-White standard errors.
Source: Author calculations. Data from BLS Quarterly Census of Employment and Wages. Tech definitions from Business Dynamics Statistics, Hecker (2005), and Goldschlag & Miranda (2020).
Studying table 4, we first note that the coefficient on $$ I\{year \geq 2020\} $$ (given by $$ \delta $$ in the regression equation) is positive and statistically significant in all specifications, indicating that annual establishment gains during the pandemic were much higher than before—by 1-1/2 to 2 log points—in non-tech industries specifically. The interaction of the time indicator with high tech variables (the coefficient $$ \beta $$) reveals that the pandemic growth boost was much larger for high tech industries than for non-tech industries. This is particularly true for the narrowest tech definition, BDS-HT, where tech industries saw a relative pandemic annual growth advantage of more than 3 log points versus non-tech industries. The third column shows that one standard deviation difference in STEM employment share is associated with three-quarters of a log point of relative establishment gains. Notably, the bottom panel reporting weighted regressions shows that the tech pandemic advantage is not simply contained to small high tech industries.
In unreported exercises we conducted several robustness checks. The regression results are similar if we include data only starting in 2017 (to avoid any potential NAICS 2012 vintage issues); the main time period effect becomes somewhat smaller but the tech interaction is largely unaffected. As noted previously, our continuous STEM share variable is missing for some industries such that the coverage of the first two columns in table 4 is slightly different from the coverage in the third column; if we run the first two regressions limiting to industries with STEM share coverage we obtain similar results. The establishment-weighted regressions reported in the bottom panel of table 4 are similar if we weight by employment instead. Results are similar if we omit the retail trade sector, where our coverage is poor due to NAICS concordance issues (explained in footnote 8). Finally, results are broadly similar if we use the second quarter or the third quarter as the reference period for the annual data (in which case, the data run through 2023:Q2 or 2023:Q3).
The year 2020 is problematic given that the Q1 reference timing in the annual data lines up with the onset of the pandemic. Indeed, it is a judgment call whether to count 2020 as part of the pandemic era or the pre-pandemic period. We count it as the pandemic era in our main regressions. If instead we omit 2020 from our regressions, the main time period effect and the tech interaction are both substantially larger.
We also consider the possibility of differing pre-pandemic trends between high tech and non-tech industries. We explore this by modifying the regression equation:
$$$$ g_{it} = \alpha_i + \delta_t + \sum_s \beta_s \cdot Tech_i \cdot I \{t=s\} + \varepsilon_{it} $$$$
In this specification, we include individual year fixed effects $$ (\delta_t) $$ and year-specific interactions with the tech indicator $$ (\beta_s) $$. Roughly speaking, the $$ \beta_s $$ coefficients provide high tech "effects" for each year. The results for regressions using the BDS-HT high tech indicator are shown in figure 2, which reports the $$ \beta_s $$ time*tech interaction coefficients as the solid lines along with 95 percent confidence intervals in dashed lines (the year 2019 interaction is omitted from the regression). The left panel shows unweighted regressions, while the right panel shows establishment-weighted regressions.
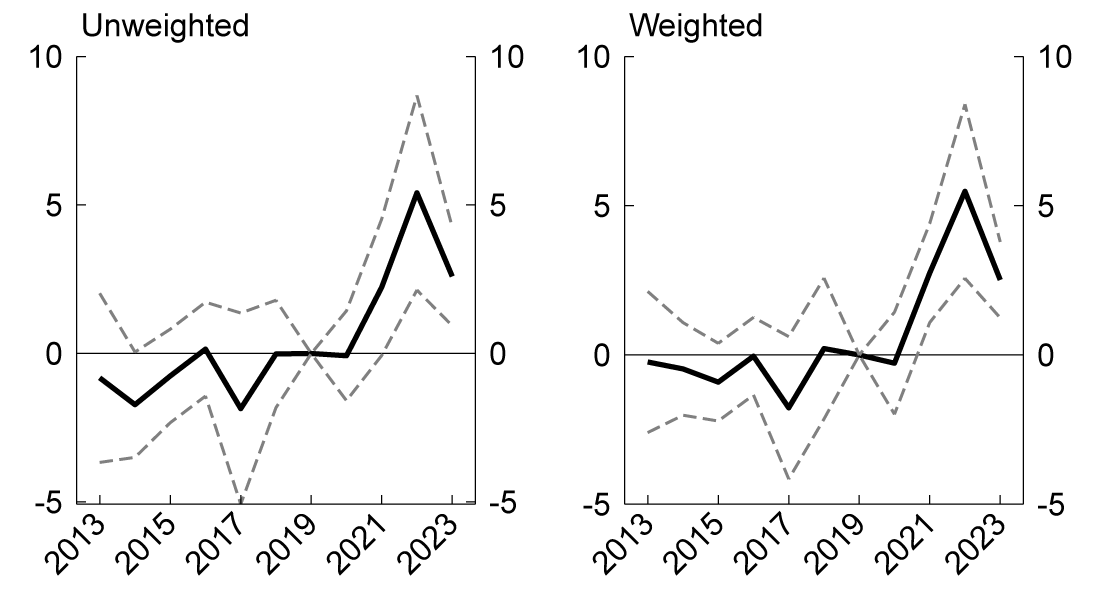
Note: Dashed lines are 95% confidence intervals. Year effect*high tech interaction coefficients.
Source: QCEW and author calculations.
Figure 2 yields several insights. First, we do not see evidence of differing pre-pandemic trends between high tech and non tech industries. Second, the post-2019 relative rise in high tech gains is strongest in 2022, though 2021 and 2023 are also strong. Third, entry was not notably strong in 2020, consistent with our note above that omitting 2020 from our main regressions strengthens the results. Note also that in Decker and Haltiwanger (2024) we find that the rise in employer entry in many measures did not start until 2021. The results shown in figure 2 are broadly similar for the Hecker (2005) and STEM share tech definitions, with the exception of the unweighted Hecker (2005)-based regression where post-pandemic coefficients are not statistically significant.
Establishment entry from incumbent versus new firms
A natural question is whether the striking pace of establishment creation in high tech industries reflects expansion of existing firms or entirely new firms engaged in entrepreneurial activity. Incumbent and new firm establishments may differ in terms of their financial constraints, productivity, size on entry, and failure risk.13 It takes some time after business application for new firms to engage in meaningful economic activity, so differences observed may reflect differences in timing. From our perspective, both types of business entry are likely important for innovation and productivity growth. In this respect, we note that incumbent firms can choose to grow through intensive (i.e., within-establishment) margins or extensive (i.e., new establishment) margins, and the extensive choice is economically meaningful (see Cao et al. 2022, Decker, McCollum, and Upton forthcoming, and Ding et al. 2022).
In the QCEW-based results we have described, we cannot observe a distinction between "greenfield" establishments opened by incumbent firms and establishments opened by entirely new entrepreneurial firms. But we can obtain suggestive evidence on this distinction from the BLS Business Employment Dynamics (BED), which is based on the same employer establishment microdata as the QCEW.14 Annual BED data allow for counting establishment births among new firms (those with age zero) versus incumbent firms, where firms are defined based on tax identifiers. Notably, these are gross establishment births, a preferable concept to the net establishment gains we analyze above in the QCEW. A tradeoff is that the BED data allowing for this distinction are available only at the broad sector level, not at the narrow level of industry detail used in our other exercises.
We first observe that establishment births surged for both incumbent and new firms during the pandemic. This can be seen in figure 3, where the left panel shows the number of establishment births for both kinds of firms over time. The right panel shows the share of total establishment births coming from incumbent firms.
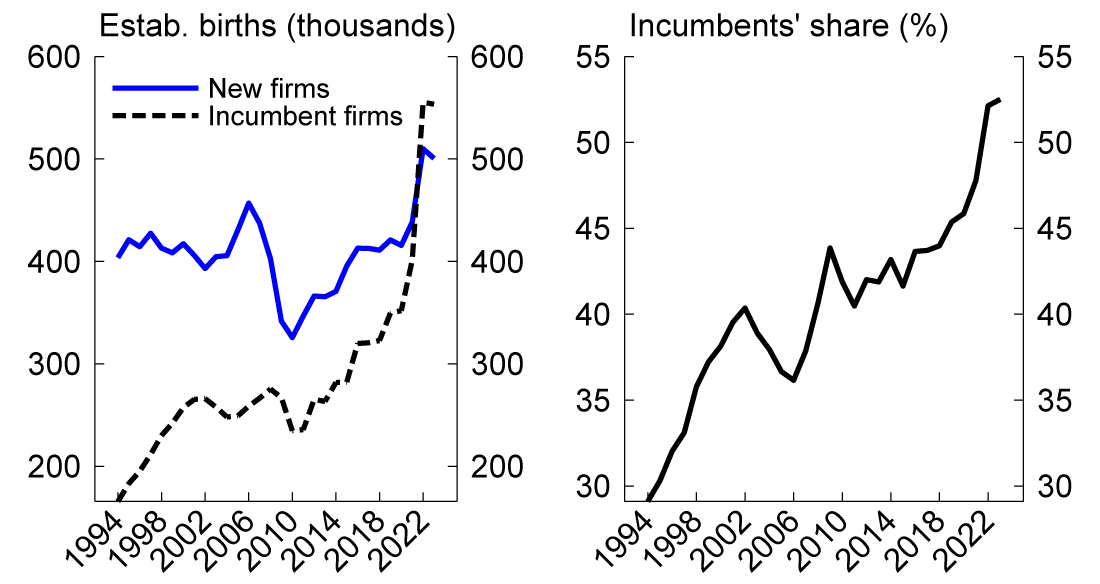
The sharp rise in establishment births during the pandemic can be seen for both new and incumbent firms, but the rise for incumbent firms is sharper. Indeed, in BED data, the share of establishment births accounted for by incumbent firms rose above half for the first time.15
Turning to the sector patterns, figure 4 features scatterplots to show the surge in establishment births for incumbent firms (vertical axes) versus new firms (horizontal axes). The left panel reports the average pace of (annual) establishment births (in thousands) during 2020 through 2023 relative to the average pace during 2013-2019. This panel helps contextualize sector-level birth surges relative to the aggregate increase, showing that the professional and business services sector, in particular, accounts for a large number of pandemic-period establishment births. Moreover, incumbent firms account for the majority of the establishment birth surge in most sectors (note that the horizontal and vertical axes have different ranges).
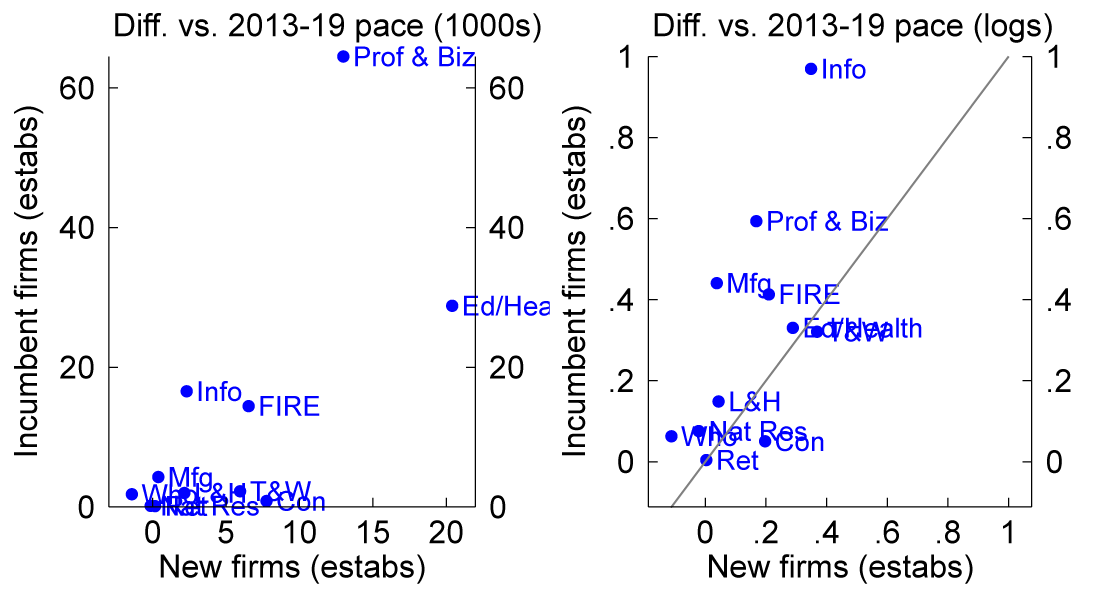
Note: 2020-2023. Establishment births. Solid line is 45-degree line. Years end in March.
Source: Business Employment Dynamics (BED).
The right panel shows the birth surge in log differences, allowing for study of within-sector growth. The solid line through the figure is a 45-degree line; this means that sectors appearing above the line saw higher sector-level establishment birth growth among incumbent firms than among new firms.
The information sector is the most tech-intensive sector, with STEM occupations accounting for 23 percent of its employment during 2013-2019 on average; professional and business services is ranked second with 18 percent, and manufacturing is third with 11 percent. Figure 4 shows that these tech-intensive sectors saw more growth of establishment births among incumbent than among new firms, though new firms also drove an elevated pace of establishment births in these sectors. The wholesale trade sector, which ranks fourth for STEM shares, is a contrasting case with only a small increase in incumbent firm establishment births and a decline in new firm births.16 We also find—not shown in the figure—that job creation by establishment births features similar patterns for these high tech sectors, with a larger rise among incumbent firms than new firms in information, professional and business services, and manufacturing.
While figure 4 might suggest a larger role for incumbent firms in the high tech entry surge after 2019, we caution that it may be too early to draw this conclusion. The data in that figure extend through early 2023. Importantly, though, business applications in BFS data remained elevated throughout 2023 and into early 2024 (with data extending through February), and applications are a forward-looking indicator in the sense that there is a lag between application and transition to full business activity.
Figure 5 reports the BFS series for predicted firm births within 8 quarters based on internal Census Bureau modeling (Bayard et al. 2018), measured as the log difference from each respective sector's 2019 average pace. The BFS predicted firm birth series can be thought of as a weighted version of the business applications data, where weights are set optimally to predict genuine employer firm births. Here we focus on the three tech-intensive sectors mentioned in association with figure 4, with one difference. The BED data in figure 4 use a very broad sector definition for the important professional and business services sector (comprised of NAICS 54, 55, and 56), but in the BFS application data we can focus more narrowly on the most tech-intensive component of that sector—professional, scientific, and technical services (NAICS 54)—in which an exceptionally high 32 percent of employment is in STEM occupations. Notably, AI-related business startups are often classified in NAICS 54 (Dinlersoz, Dogan, and Zolas 2024).
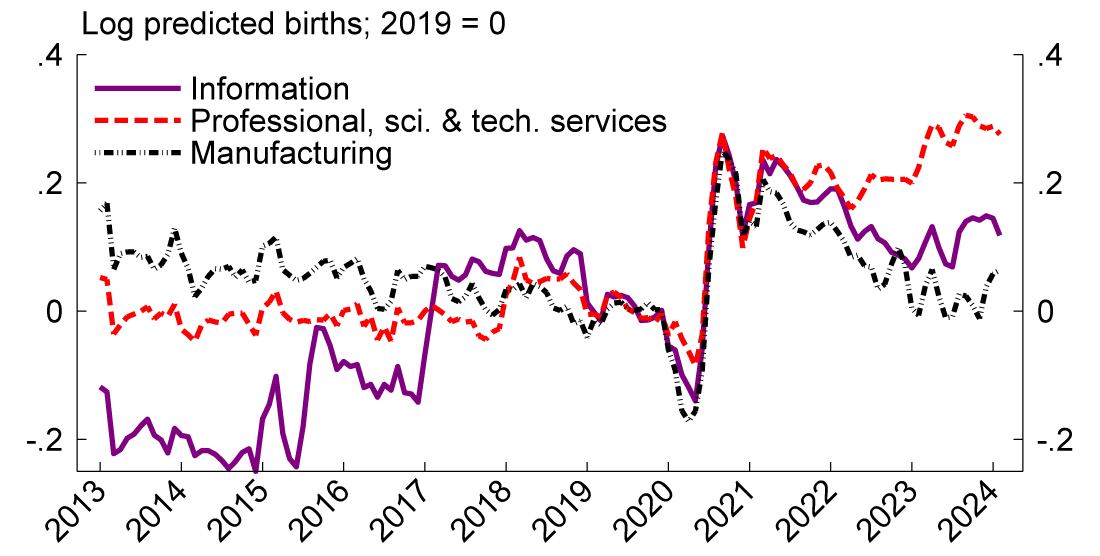
Note: Log 8-quarter predicted firm births (PBF8Q) relative to 2019 average. Seasonally adjusted. 3-month moving average.
Source: Business Formation Statistics.
Figure 5 shows that predicted firm births in professional, scientific and technical services surged in the pandemic and have remained elevated all the way into early 2024. Notably, this sector includes some of the high tech industries highlighted in tables 1 and 2 above, such as scientific research and development services, computer systems design, and scientific and technical consulting. The information sector also saw a large surge in application-predicted firm births early in the pandemic; this surge has receded some, though predicted births were still historically elevated in the most recent data. Manufacturing, on the other hand, now appears to have returned to pre-pandemic norms.
Figure 5 suggests that business entry from new firms—and not just incumbent firms—continues to be elevated in key tech-intensive sectors. The early 2024 statistics reflect predicted firm births over the next 8 quarters, indicating that new firm entry is likely to remain elevated at least until 2026. Therefore, over time, the relative roles of new and incumbent firms in the pandemic high tech entry surge could evolve further.
Conclusion
The surge in pandemic business entry appears to have featured a significant high tech component. Tech industries saw a larger rise in net establishment entry than did non-tech industries and account for a disproportionate share of the increase in the number of U.S. business establishments since 2019. Preliminary evidence suggests that incumbent firms may have been particularly active in making high tech investments through new establishment creation—with notable associated job creation—though new entrepreneurial firms made notable contributions as well and appear likely to continue doing so.
References
Alon, Titan, David Berger, Rob Dent, and Benjamin Pugsley. 2018. "Older and slower: The startup deficit's lasting impact on productivity growth." Journal of Monetary Economics 93 (January): 1102-47.
Bayard, Kimberly, Emin Dinlersoz, Timothy Dunne, John Haltiwanger, Javier Miranda, and John Stevens. "Early-stage business formation: An analysis of applications for employer identification numbers." NBER working paper no. 24364.
Cao, Dan, Henry Hyatt, Toshihiko Mukoyama, and Erick Sager. 2022. "Firm growth through new establishments." Working paper. At https://www.ericksager.com/uploads/3/8/0/3/3803061/chms.pdf.
Decker, Ryan and John Haltiwanger. 2024. "Surging business formation in the pandemic: Causes and consequences?" Fall 2023 Brookings Papers on Economic Activity. Forthcoming.
Decker, Ryan, John Haltiwanger, Ron Jarmin, and Javier Miranda. 2014. "The role of entrepreneurship in US job creation and economic dynamism." Journal of Economic Perspectives 28 no. 3:3-24.
Decker, Ryan, John Haltiwanger, Ron Jarmin, and Javier Miranda. 2016. "Where has all the skewness gone? The decline in high-growth (young) firms in the U.S." European Economic Review 86 (July): 4-23.
Decker, Ryan, Meagan McCollum, and Gregory Upton, Jr. Forthcoming. "Boom town business dynamics." Journal of Human Resources.
Ding, Xiang, Teresa Fort, Stephen Redding, and Peter Schott. 2022. "Structural change within versus across firms: Evidence from the United States." Working paper. At https://georgetown.app.box.com/s/kehl0f671n9la2hn784ahsfdmu5cuoea.
Dinlersoz, Emin, Can Dogan, and Nikolas Zolas. 2024. "Startup up AI." Center for Economic Studies working paper no. CES 24-09.
Fernald, John. 2015. "Productivity and potential output before, during, and after the Great Recession." NBER Macroeconomics Annual 2014 29 no. 1:1-51.
Foster, Lucia, Cheryl Grim, John Haltiwanger and Zoltan Wolf. 2021. "Innovation, Productivity Dispersion, and Productivity Growth" in Measuring and Accounting for Innovation in the Twenty-First Century, (Corrado, Haskel, Miranda, and Sichel, eds.) NBER/University of Chicago Press.
Goldschlag, Nathan and Javier Miranda. 2020. "Business dynamics of high tech industries." Journal of Economics & Management Strategy 29 no. 1:3-30.
Haltiwanger, John, Ian Hathaway, and Javier Miranda. 2014. "Declining business dynamism in the U.S. high-technology sector." The Kauffman Foundation.
Hecker, Daniel. 2005. "High-technology employment: A NAICS-based update." Monthly Labor Review 128 no. 7:57-72.
1. Decker: Division of Research and Statistics at the Federal Reserve Board. Haltiwanger: University of Maryland and NBER. Without implication, we thank Seth Murray for helpful comments and suggestions. The analysis and conclusions set forth are those of the authors and do not indicate concurrence by other members of the research staff or the Board of Governors of the Federal Reserve System. Return to text
2. In statistical agency parlance, a "establishment" is a business operating location with at least one formal employee. A "firm" is a collection of one or more establishments under a common tax identifier (in BLS data) or under common ownership or operational control (in Census Bureau data). Return to text
3. The first draft of the cited paper dates to fall of 2022. Return to text
4. As we discussed in Decker and Haltiwanger (2024), applications for new employer businesses are designed, by construction, to predict new firm entry rather than establishment entry for existing businesses. An existing business does not need a new EIN to open a new establishment. However, it is not surprising that new firm entry and establishment entry of existing businesses are closely related. Return to text
5. The information sector is NAICS 51. The professional and business services sector is NAICS 54, 55, and 56 combined. Return to text
6. The obvious drawback of being limited to net, rather than gross, establishment entry is that fluctuations in establishment exit (death) could complicate interpretation of our results; economywide establishment exit spiked during 2020:Q2 but then was fairly low for a time, rising some during 2021 and moving up robustly in mid-2022. That said, net establishment entry is worth studying on its own as an indicator of the aggregate strength of high tech investments and associated likely productivity implications. Return to text
7. A first-quarter reference period is consistent with other annual business dynamics data, though we find very similar results using the second quarter or the third quarter as the reference period. We acknowledge that the BLS does not intend for the QCEW to be a time series data product. Nevertheless, it is used as such in many applications, including the U.S. national accounts. We proceed with studying the QCEW as a time series while paying careful attention to changes in industry classification and scope that could compromise the time series. Return to text
8. During this time period there are three NAICS vintages in the data: NAICS 2012, 2017, and 2022. We first harmonize 4-digit codes that experience one-to-one mappings across vintages, of which there are two (the NAICS 4571 and 5132 industries in NAICS 2022 are 4471 and 5112, respectively, in NAICS 2017). We then aggregate certain sets of 4-digit codes to the 3-digit level to match with our high tech measures (which we describe further below); these codes are 327, 484, 517, 523, and 531. We drop NAICS 5211 (monetary authorities – central bank) because it shows an implausible rise in establishment counts, possibly due to erroneous self reporting of industry codes in the QCEW's unemployment insurance system source data. We drop NAICS 8141 (private households) due to an apparent large scope change in 2013. In NAICS 6241 (individual and family services), there is an apparent scope change in 2013 within the 5-digit industry 62412 (services for the elderly and persons with disabilities); we reconstruct 6241 by combining its constituent 5-digit industries excluding 62412. After these adjustments, a total of 311 4-digit NAICS codes appear in the data for 2013-2023; 43 of the 311 codes do not cross all three NAICS vintages and cannot be cleanly harmonized, and 7 of the 311 codes experience scope changes between NAICS 2017 and NAICS 2022 that cannot be easily cleaned. The vast majority of scope and code change difficulties are related to NAICS 454 (nonstore retailers), which appears in NAICS 2012 and NAICS 2017 but was discontinued for NAICS 2022, when various nonstore retail categories were distributed to be categorized with their store-based counterpart industries (e.g., to combine nonstore and in-store shoe retailers). Because most narrow industries within the retail trade sector have some scope relationship to NAICS 454 in this transition, we lose most of this sector in our analysis—an unfortunate effect of this NAICS classification change (and a highly consequential effect, given the importance of NAICS 454 in the pandemic entry surge as documented by Decker and Haltiwanger 2024 and related work). While our balanced panel of industries accounts for roughly 90 percent of 2019 QCEW establishments and employment, it only accounts for 15 percent of the retail trade sector. Importantly, poor coverage of establishments in retail trade does not imply that the activities of large retail firms are missing; for example, a large retailer could open a new establishment that operates in an industry for which we do have coverage. We also lose some industries in information, where we retain 70 percent of establishments and employment, and in other services (due to 8141 mentioned above), where we retain 70 percent of establishments and 95 percent of employment. Return to text
9. See Goldschlag and Miranda (2020) for a discussion of various ways to measure industry high tech intensity, including a discussion of use of research and development expenditures. Return to text
10. The BDS-HT industries are those NAICS 2017 industries in which the STEM employment share is at least five times the national average across industries during 2007 through 2017; these industries are 3341, 3342, 3344, 3345, 3364, 5112, 5182, 5191, 5413, 5415, and 5417. Return to text
11. The Hecker (2005) industries are those with STEM employment share at least twice the average in 2002; see Goldschlag and Miranda (2020) Appendix A for a full list of Hecker (2005) high tech codes (we use all three levels). Return to text
12. Unsurprisingly, at the industry level, establishment gains and employment growth are positively correlated. In particular, if we measure establishment gains and employment growth in the pandemic versus pre-pandemic period at the industry level, the unweighted correlation between establishment gains and employment is around 0.7. The establishment-weighted correlation is around 0.45, and the employment-weighted correlation is around 0.5. In other words, industries that saw a large surge in net establishment entry during the pandemic era tended to also see a large surge in employment growth. Return to text
13. In Census Bureau Business Dynamics Statistics, establishment births associated with incumbent firms tend to be more than twice as large as new firm establishment births: during 2013-2019 on average, incumbent firm establishment births average 11.4 employees while new firm establishments average 5.3 employees. Establishment exit rates are declining in firm age; for 2013-2019 on average, the establishment exit rate for new firms was 24 percent and declined monotonically with firm age, falling below 6 percent for firms older than 25. Return to text
14. For these exercises we use the BED "research" data that provide establishment counts and job flows by establishment and firm age. Establishment births among incumbent firms are equal to total establishments with age zero minus the establishments of firms with age zero. See the appendix to Decker and Haltiwanger (2024) for discussion of firm identifiers in BED data versus Census Bureau data. Return to text
15. Alternative data on the incumbent share of establishment births from the Census Bureau in the Business Dynamics Statistics, which only run through 2021, show a similar trend to the BED data prior to 2010 but a sharp divergence after 2010, when the BDS incumbent share is low relative to BED. This is an important measurement discrepancy requiring further study. Return to text
16. We omit the utilities sector from this ranking, as it is not present in the BED data. If we included utilities it would rank third for tech intensity, so manufacturing and wholesale trade would rank fourth and fifth, respectively. Return to text
Decker, Ryan A., and John Haltiwanger (2024). "High tech business entry in the pandemic era," FEDS Notes. Washington: Board of Governors of the Federal Reserve System, April, 19, 2024, https://doi.org/10.17016/2380-7172.3499.
Disclaimer: FEDS Notes are articles in which Board staff offer their own views and present analysis on a range of topics in economics and finance. These articles are shorter and less technically oriented than FEDS Working Papers and IFDP papers.